Branding the role of OR in AI with the Support of
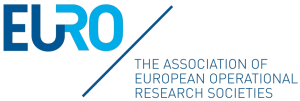
Machine Learning NeEDS Mathematical Optimization is an online seminar series, organized by Emilio Carrizosa (IMUS – Instituto de Matemáticas de la Universidad de Sevilla) and Dolores Romero Morales (CBS – Copenhagen Business School) with the collaboration of PhD Students Nuria Gómez-Vargas (IMUS) and Thomas Halskov (CBS). We also thank the past collaboration of Dr Cristina Molero-Río (former PhD student at IMUS) and PhD Students Kseniia Kurishchenko (CBS) and Jasone Ramírez-Ayerbe (IMUS). One of the goals of this activity is to brand the role of Operations Research in Artificial Intelligence with the support of EURO.
This series includes a number of presentations from leading academics in the field of Data Science and Analytics that will cover important topics such as explainability, fairness, fraud, privacy, etc. Mathematical Modeling and Mathematical Optimization will be at the core of their presentations. We also have the YOUNG Online Seminar Series “Machine Learning NeEDS Mathematical Optimization”. In each YOUNG session, three junior academics will show their latest results in this burgeoning area .
The format is a weekly session that takes place every Monday, at 16.30 (CET), and has been active since January 11, 2021. It is 100% online-access, and it has speakers from around the globe. The Online Seminar Series is free thanks to the funding the EU gives to our H2020 MSCA NeEDS project, as well as the support given by Universidad de Sevilla (US) and Copenhagen Business School (CBS). This support is gratefully acknowledged.
Shortly after each seminar, its video recording will be available at the NeEDS YouTube channel.
We would like to thank the more than 100 speakers, the more than 2000 colleagues registered to our mailing list, and EURO.
The OSS was created within the EU-funded project NeEDS, which is closing after the summer. Hence, the series of talks for the next academic year will have a different name, and if we are lucky the new seminar series will have a more institutional support from our community. We will keep you posted on this through our mailinglist and social networks. We have been working on a new round of talks for the next academic year. The list of confirmed senior speakers can be found at the bottom of this email.
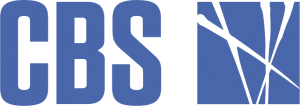
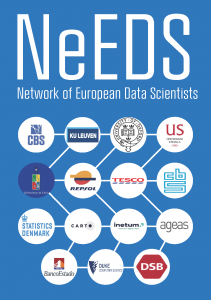
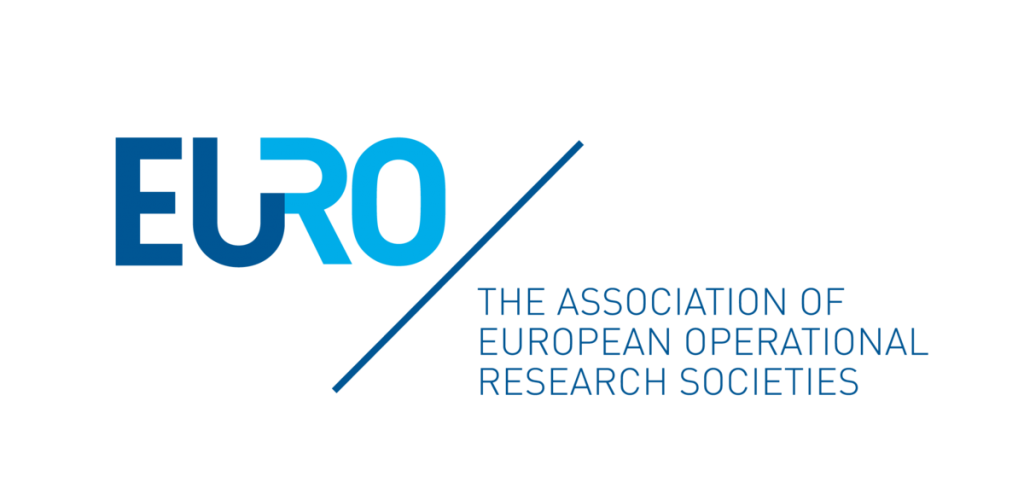
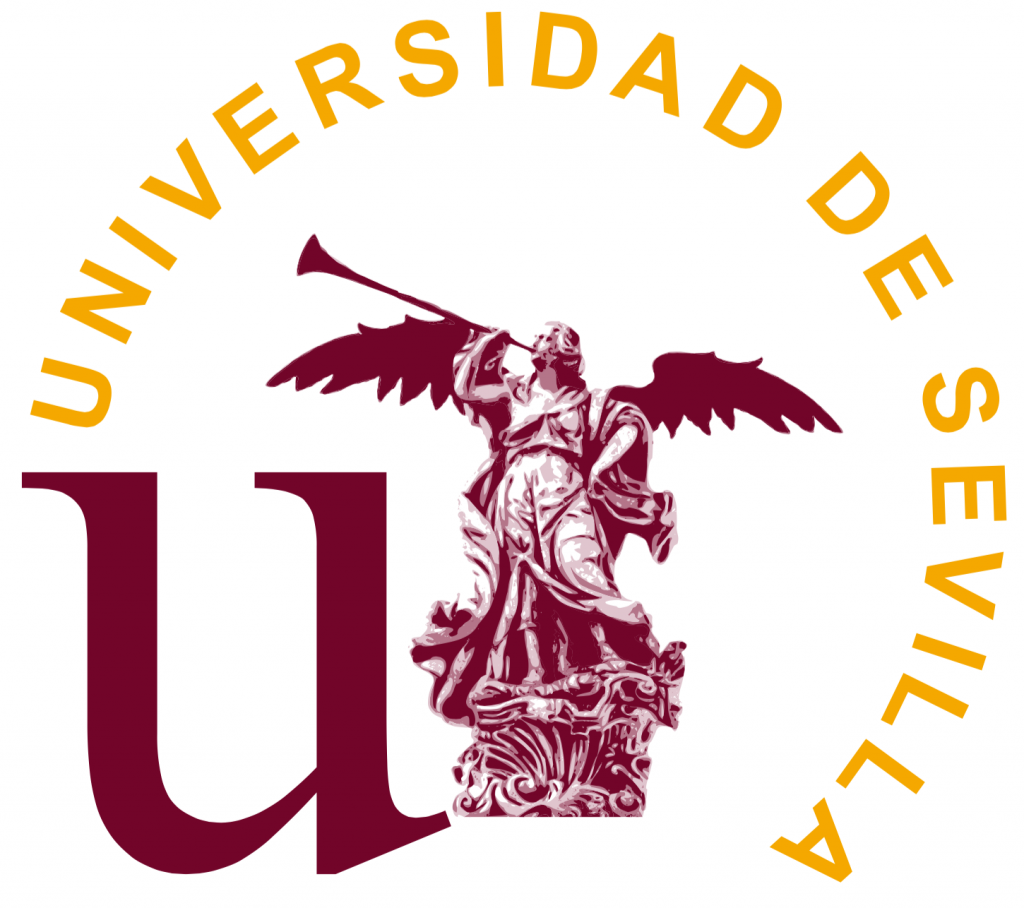
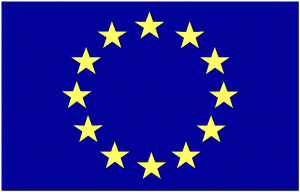
Click below for Talks of Previous Seasons
February 12, 2024, 16.30 – 17.30 (CET)
Decentralized Bilevel Optimization
To attend the seminar, click here.
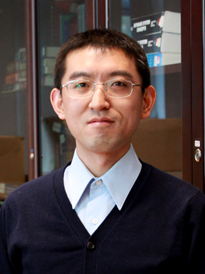
Speaker: Prof Shiqian Ma
Associate Professor
Department of Computational Applied Math and Operations Research
Department of Electrical and Computer Engineering
Rice University
USA
Abstract: Bilevel optimization has received tremendous attention recently due to its great success in machine learning applications such as meta learning, reinforcement learning, and hyperparameter optimization. In this talk, we discuss recent advances on bilevel optimization algorithms in decentralized networks. We will address the issues of how to estimate the hypergradient in decentralized networks, how to design single-loop algorithms, and how to improve the per-iteration complexity using moving average techniques, etc.
February 19, 2024, 16.30 – 17.30 (CET)
Risk Quadrangle and Applications: Support Vector Regression (SVR)
To attend the seminar, click here.
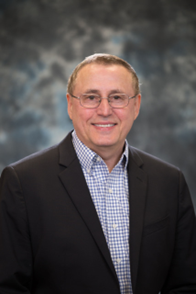
Speaker: Prof Stan Uryasev
Professor and Frey Family Endowed Chair of Quantitative Finance
Stony Brook University
USA
Abstract: The Support Vector Regression (SVR) is investigated in the Risk Quadrangle framework. ε-SVR and ν-SVR, correspond to the minimization of equivalent error measures (Vapnik error and CVaR norm) with a regularization. These two error measures, define corresponding Risk Quadrangles. We show that SVR is an asymptotically unbiased estimator of the average of two symmetric conditional quantiles. Equivalence of ε-SVR and ν-SVR follows from the quadrangle framework. SVR is formulated as a deviation minimization with a regularization penalty by applying Error Shaping Decomposition of Regression. Moreover, f-Divergence Quadrangle implies that SVR is a robust variant of the mean-absolute regression with regularization. Finally, the dual formulation of SVR is derived.
February 26, 2024, 16.30 – 17.30 (CET)
Learning and Optimization: Separate or Integrate?
To attend the seminar, click here.
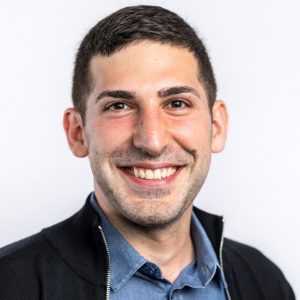
Speaker: Prof Nathan Kallus
Associate Professor at Cornell Tech, Cornell University
Research Director at Machine Learning & Inference Research, Netflix
USA
Abstract: Predictive side information (aka context) makes optimization under uncertainty less uncertain, but leveraging it requires we learn a potentially complex predictive relationship. We might use off-the-shelf ML methods for this, but their training process ignores the downstream optimization task where the model would be plugged in. Instead, we can train the model in an end-to-end fashion to optimize the downstream costs of the decisions it would induce. In this talk I will tackle the question, which is better? That is, should we separate or integrate the learning and optimization tasks? I show that in linear problems, where we only care to learn the mean-prediction (aka regression) function, the naive separated approach surprisingly has regret that vanishes orders faster than end-to-end methods — a consequence of real problems not having arbitrarily bad near-dual-degeneracy. However, for general (nonlinear) contextual optimization, which involves the hard-to-learn conditional-probability-prediction function, it may be better to bypass learning this complex object by integrating the tasks and directly learning a decision policy. I show how to do this tractably near-optimally for tree-based policies and build scalable decision forests using approximate splitting criteria based on second-order optimization perturbation analysis.
March 4, 2024, 16.30 – 17.30 (CET)
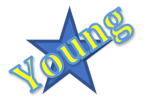
To attend the seminars, click here.
Learning Optimal Classification Trees Robust to Distribution Shifts
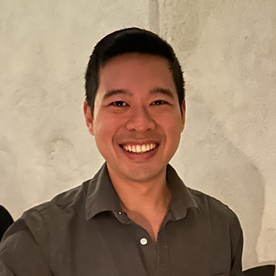
Abstract: We consider the problem of learning classification trees that are robust to distribution shifts between training and testing/deployment data. This problem arises frequently in high stakes settings such as public health and social work where data is often collected using self-reported surveys which are highly sensitive to e.g., the framing of the questions, the time when and place where the survey is conducted, and the level of comfort the interviewee has in sharing information with the interviewer. We propose a method for learning optimal robust classification trees based on mixed-integer robust optimization technology. In particular, we demonstrate that the problem of learning an optimal robust tree can be cast as a single-stage mixed-integer robust optimization problem with a highly nonlinear and discontinuous objective. We reformulate this problem equivalently as a two-stage linear robust optimization problem for which we devise a tailored solution procedure based on constraint generation. We evaluate the performance of our approach on numerous publicly available datasets, and compare the performance to a regularized, non-robust optimal tree. We show an increase of up to 12.48% in worst-case accuracy and of up to 4.85% in average-case accuracy across several datasets and distribution shifts from using our robust solution in comparison to the non-robust one.
Can machine learning methods effectively model travel mode choice? Beyond predictive performance
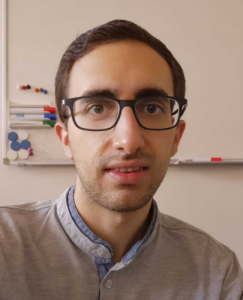
Speaker 2: José Ángel Martín Baos
Assistant Professor
Department of Mathematics
University of Castilla-La Mancha
Spain
Abstract: This talk explores the selection of machine learning models for travel mode choice prediction, emphasizing factors beyond predictive performance, including interpretability, computational complexity, and data efficiency. It addresses limitations in existing research by systematically comparing various models across multiple aspects, revealing that models with the highest predictive performance in the literature (namely Gradient Boosting and Random Forests) often provide poorer estimates of behavioral indicators and aggregate market shares compared to alternatives like Deep Neural Networks and Multinomial Logit (MNL). The MNL model is found to perform robustly across various scenarios, although the use of machine learning techniques can help enhance estimates of behavioral indicators such as willingness to pay.
Learning the Follower’s Objective Function in Sequential Bilevel Games
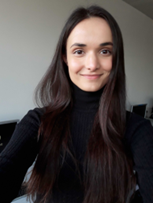
Abstract: In this talk we consider bilevel optimization problems in which the leader has no or only partial knowledge about the objective function of the follower. The studied setting is a sequential one in which the bilevel game is played repeatedly. This allows the leader to learn the objective function of the follower over time. We focus on two methods: a multiplicative weight update (MWU) method and one based on the lower-level’s KKT conditions that are used in the fashion of inverse optimization. The MWU method requires less assumptions but the convergence guarantee is also only on the objective function values, whereas the inverse KKT method requires stronger assumptions but actually allows to learn the objective function itself. The applicability of the proposed methods is shown using a sequential bilevel pricing game in which the leader needs to learn the utility function of the follower.
March 11, 2024, 16.30 – 17.30 (CET)
Role of Analytics Professionals in Building a Data Driven Culture
To attend the seminar, click here.
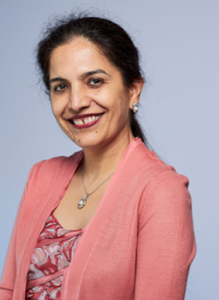
Abstract: This talk is going to focus on the value of Data Ecosystems, Starting with the business outcome, Composition of teams, and evolving skills needed to take Algorithms in R&D to Commercial Reality and building a Data Driven Culture and creating impact.
March 18, 2024, 16.30 – 17.30 (CET)
FunSearch: Discovering new mathematics and algorithms using Large Language Models
To attend the seminar, click here.
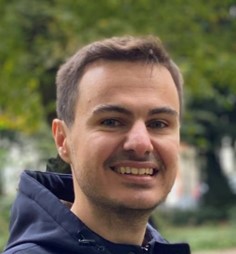
Abstract:
In this talk I will present FunSearch, a method to search for new solutions in mathematics and computer science. FunSearch works by pairing a pre-trained LLM, whose goal is to provide creative solutions in the form of computer code, with an automated “evaluator”, which guards against hallucinations and incorrect ideas. By iterating back-and-forth between these two components, initial solutions “evolve” into new knowledge. I will present the application of FunSearch to a central problem in extremal combinatorics — the cap set problem — where we discover new constructions of large cap sets going beyond the best known ones, both in finite dimensional and asymptotic cases. This represents the first discoveries made for established open problems using LLMs. Then, I will present the application of FunSearch to an algorithmic problem, online bin packing, which showcases the generality of the method. In this use case, FunSearch finds new heuristics that improve upon widely used baselines. I will conclude the talk by discussing the implications of searching in the space of code.
April 8, 2024, 16.30 – 17.30 (CET)
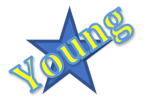
To attend the seminars, click here.
Factor Model of Mixtures
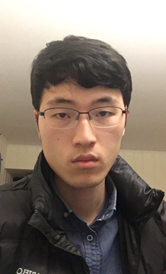
Speaker 1: Cheng Peng
PhD student at Department of Applied Mathematics & Statistics
Stony Brook University
USA
Abstract: This paper proposes a new approach to estimating the distribution of a response variable conditioned on observing some factors. The proposed approach possesses desirable properties of flexibility, interpretability, tractability and extendability. The conditional quantile function is modeled by a mixture (weighted sum) of basis quantile functions, with the weights depending on factors. The estimation problem is formulated as a convex optimization problem. It can be viewed as conducting quantile regressions for all confidence levels simultaneously while avoiding quantile crossing by design. The estimation problem is equivalent to minimizing the continuous ranked probability score (CRPS). If time allows, we will also demonstrate its potential application in classification, ensemble modeling and breakpoint detection.
Estimate-Then-Optimize versus Integrated-Estimation-Optimization versus Sample Average Approximation: A Stochastic Dominance Perspective
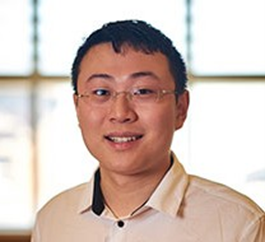
Speaker 2: Haofeng Zhang
PhD student
Department of Industrial Engineering and Operations Research
Columbia University
New York
USA
Abstract: In data-driven stochastic optimization, model parameters of the underlying distribution need to be estimated from data in addition to the optimization task. Recent literature considers integrating the estimation and optimization processes by selecting model parameters that lead to the best empirical objective performance. This integrated approach, which we call integrated-estimation-optimization (IEO), can be readily shown to outperform simple estimate-then-optimize (ETO) when the model is misspecified. In this paper, we show that a reverse behavior appears when the model class is well-specified and there is sufficient data. Specifically, for a general class of nonlinear stochastic optimization problems, we show that simple ETO outperforms IEO asymptotically when the model class covers the ground truth, in the strong sense of stochastic dominance of the regret. Namely, the entire distribution of the regret, not only its mean or other moments, is always better for ETO compared to IEO. Our results also apply to constrained, contextual optimization problems where the decision depends on observed features. Whenever applicable, we also demonstrate how standard sample average approximation (SAA) performs the worst when the model class is well-specified in terms of regret, and best when it is misspecified. Finally, we provide experimental results to support our theoretical comparisons and illustrate when our insights hold in finite-sample regimes and under various degrees of misspecification.
Unboxing Tree Ensembles for interpretability: a hierarchical visualization tool and a multivariate optimal re-built tree
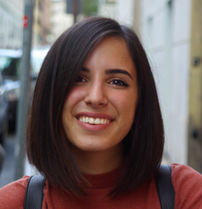
Speaker 3: Giulia Di Teodoro
PhD in the Department of Computer, Control and Management Engineering Antonio Ruberti (DIAG)
Sapienza University of Rome
Italy
Abstract: The interpretability of models has become a crucial issue in Machine Learning because of algorithmic decisions’ growing impact on real-world applications. Tree ensemble methods, such as Random Forests or XgBoost, are powerful learning tools for classification tasks. However, while combining multiple trees may provide higher prediction quality than a single one, it sacrifices the interpretability property resulting in «black-box» models. In light of this, we aim to develop an interpretable representation of a tree-ensemble model that can provide valuable insights into its behavior. First, given a target tree-ensemble model, we develop a hierarchical visualization tool based on a heatmap representation of the forest’s feature use, considering the frequency of a feature and the level at which it is selected as an indicator of importance. Next, we propose a mixed-integer linear {programming (MILP)} formulation for constructing a single optimal multivariate tree that accurately mimics the target model predictions. The goal is to provide an interpretable surrogate model based on oblique hyperplane splits, which uses only the most relevant features according to the defined forest’s importance indicators. {The MILP model includes a penalty on feature selection based on their frequency in the forest to further induce sparsity of the splits.} The natural formulation has been strengthened to improve the computational performance of {mixed-integer} software. Computational experience is carried out on benchmark datasets from the UCI repository using a state-of-the-art off-the-shelf solver. Results show that the proposed model is effective in yielding a shallow interpretable tree approximating the tree-ensemble decision function.
April 22, 2024, 16.30 – 17.30 (CET)
Integrating Stochastic Optimization and Machine Learning via Residuals
To attend the seminar, click here.
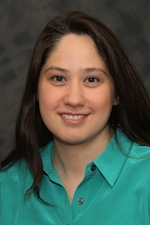
Speaker: Prof Güzin Bayraksan
Professor and Associate Chair for Research in the Integrated Systems Engineering Department
Affiliated faculty member of the Sustainability Institute and the Translational Data Analytics Institute at the Ohio State University
The Ohio State University
USA
Abstract: We consider data-driven approaches that integrate a machine learning prediction model within stochastic optimization, given joint observations of uncertain parameters and covariates. Given a new covariate observation, the goal is to choose a decision that minimizes the expected cost conditioned on this observation. We first present a Sample Average Approximation (SAA) approach for approximating this problem that incorporates residuals from the learning step. Then, in the limited-data regime, we consider Distributionally Robust Optimization (DRO) variants of these models. Our framework is flexible in the sense that it can accommodate a variety of learning setups and DRO ambiguity sets. We investigate the asymptotic and finite sample properties of SAA and the DRO variants obtained using Wasserstein, sample robust optimization, and phi-divergence-based ambiguity sets. We discuss extensions to decision-dependent settings and present applications using real-world data.
April 29, 2024, 16.30 – 17.30 (CET)
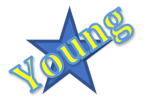
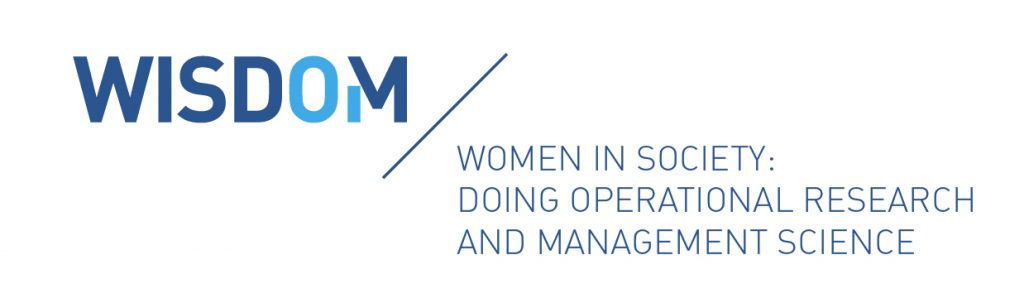
To attend the seminars, click here.
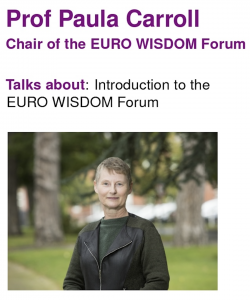
A predict-and-optimize approach to profit-driven churn prevention
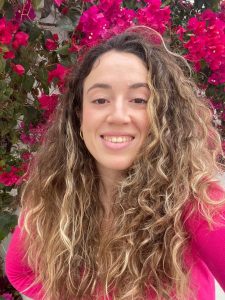
Speaker 1: Nuria Gómez-Vargas
PhD student at Department of Statistics and Operations Research
University of Seville
Spain
Abstract: In this work, we introduce a novel predict-and-optimize method for profit-driven churn prevention. We frame the task of targeting customers for a retention campaign as a regret minimization problem. The main objective is to leverage individual customer lifetime values (CLVs) to ensure that only the most valuable customers are targeted. In contrast, many profit-driven strategies focus on churn probabilities while considering average CLVs. This often results in significant information loss due to data aggregation. Our proposed model aligns with the guidelines of Predict-and-Optimize (PnO) frameworks and can be efficiently solved using stochastic gradient descent methods.
Mixed-Integer Quadratic Optimization and Iterative Clustering Techniques for Semi-Supervised Support Vector Machines
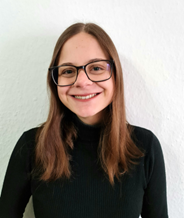
Abstract: Among the most famous algorithms for solving classification problems are support vector machines (SVMs), which find a separating hyperplane for a set of labeled data points. In some applications, however, labels are only available for a subset of points. Furthermore, this subset can be non-representative, e.g., due to self-selection in a survey. Semi-supervised SVMs tackle the setting of labeled and unlabeled data and can often improve the reliability of the results. Moreover, additional information about the size of the classes can be available
from undisclosed sources. We propose a mixed-integer quadratic optimization (MIQP) model that covers the setting of labeled and unlabeled data points as well as the overall number of points in each class. Since the MIQP’s solution
time rapidly grows as the number of variables increases, we introduce an iterative clustering approach to reduce the model’s size. Moreover, we present an update rule for the required big-M values, prove the correctness of the iterative clustering method as well as derive tailored dimension-reduction and warm-starting techniques. Our numerical results show that our approach leads to a similar accuracy and precision than the MIQP formulation but at much lower computational cost. Thus, we can solve larger problems. With respect to the original SVM formulation, we observe that our approach has even better accuracy and precision for biased samples.
Distributionally Robust Linear Quadratic Control
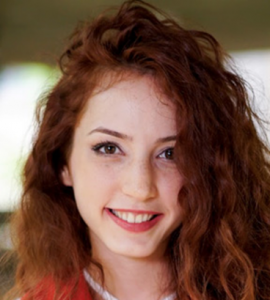
Speaker 3: Bahar Taskesen
Ph.D. candidate
Risk Analytics and Optimization Lab
École Polytechnique Fédérale de Lausanne (EPFL)
Switzerland
Abstract: Linear-Quadratic-Gaussian (LQG) control is a fundamental control paradigm that is studied in various fields such as engineering, computer science, economics, and neuroscience. It involves controlling a system with linear dynamics and imperfect observations, subject to additive noise, with the goal of minimizing a quadratic cost function for the state and control variables. In this work, we consider a generalization of the discrete-time, finite-horizon LQG problem, where the noise distributions are unknown and belong to Wasserstein ambiguity sets centered at nominal (Gaussian) distributions. The objective is to minimize a worst-case cost across all distributions in the ambiguity set, including non-Gaussian distributions. Despite the added complexity, we prove that a control policy that is linear in the observations is optimal for this problem, as in the classic LQG problem. We propose a numerical solution method that efficiently characterizes this optimal control policy. Our method uses the Frank-Wolfe algorithm to identify the least-favorable distributions within the Wasserstein ambiguity sets and computes the controller’s optimal policy using Kalman filter estimation under these distributions.
May 6, 2024, 16.30 – 17.30 (CET)
Online learning and decision-making for renewables participating in electricity markets
To attend the seminar, click here.
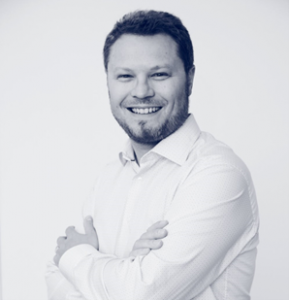
Speaker: Prof Pierre Pinson
Chair of Data-Centric Design Engineering at Imperial College London, Dyson School of Design Engineering, United Kingdom
Chief Scientist at Halfspace, Denmark
Technical University of Denmark, Department of Technology, Management and Economics, Denmark
Abstract: There is extensive literature on the analytics involved in the participation of renewable energy producers in electricity markets, covering both forecasting and decision-making. In their simplest form, participation strategies are to be seen as newsvendor problems (taking a decision-making perspective), or quantile regression problems (if taking a forecasting perspective instead). We will therefore explore recent advances at the interface between learning, forecasting and stochastic optimisation of relevance to renewable energy producers participating in electricity markets. This will cover online learning and decision-making, as well as distributionally robust optimisation.
Talks of Season VI
October 2, 2023, 16.30 – 17.30 (CET)
Outcome Reasoning: the under-discussed engine powering black box development
To attend the seminar, click here.
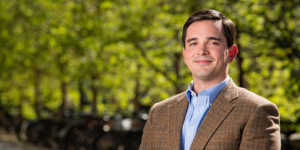
Speaker: Prof Mike Baiocchi
Associate Professor
Department of Epidemiology and Population Health
Stanford University
USA
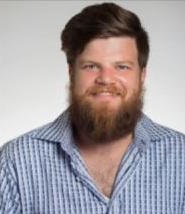
Abstract: For a long time in (bio)statistics we only had two fundamental ways of reasoning using data: warranted reasoning (e.g., randomized trials) and model reasoning (e.g., linear models). In the 1980s a new, extraordinarily productive way of reasoning about algorithms emerged: «outcome reasoning.» Outcome reasoning has come to dominate areas of data science, it has been under-discussed and its impact under-appreciated. For example, it is the primary way we reason about «black box» algorithms. In this talk we will discuss its current use (i.e., as «the common task framework») and its limitations. I will show why we find a large class of prediction-problems are inappropriate for this new type of reasoning. I will then discuss a way to extend this type of reasoning for use, where appropriate, in assessing algorithms for deployment (i.e., when using a predictive algorithm «in the real world»). We purposefully developed this new framework so both technical and non-technical people can discuss and identify key features of their prediction problem.
October 9, 2023, 16.30 – 17.30 (CET)
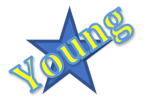
To attend the seminars, click here.
A Graph Neural Network-based Reduce-then-Optimize Heuristic for the Fixed-Charge Transportation Problem
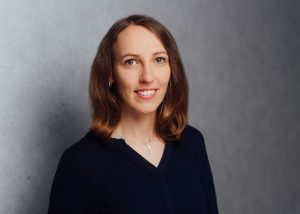
Speaker 1: Caroline Spieckermann
PhD Candidate
Logistics and Supply Chain Management, TUM School of Management, Technical University of Munich, Munich, Germany
Advanced Optimization in a Networked Economy (GRK 2201), Technical University of Munich, Munich, Germany
Abstract: The Fixed-Charge Transportation Problem (FCTP) is a well-known combinatorial optimization problem whose NP-hardness requires the application of efficient solution algorithms. We present a machine learning-based heuristic that reduces the problem complexity by predicting a relevant subset of edges. The reduced problem can be solved efficiently with any suitable exact or heuristic solver. By using machine learning only for preprocessing the problem and resorting to a high-quality solver for the subsequent optimization, we effectively utilize the distinct strengths of machine learning and combinatorial optimization. We base the edge predictor on a bipartite graph neural network (GNN) that is both parameter-efficient and problem size-agnostic. We evaluate the performance on various FCTP benchmark data sets to analyze the impact of different structural properties, such as the supply-demand-ratio or the predominance of the fixed costs, on the problem complexity and edge predictability. The GNN shows good prediction and generalization capabilities that translate into high quality solutions across all data sets (< 0.5% optimality gaps) while runtimes of a state-of-the-art MILP solver are reduced by 80-95%. When a heuristic solver is used or runtime is limited, the problem reduction can systematically improve the solver performance by providing an effective reduction of the search space. While different problem characteristics do have an impact on the problem difficulty, we find that they hardly affect the edge predictability, making the approach applicable for a broad range of FCTPs.
Reliable Adaptive Stochastic Optimization with High Probability Guarantees
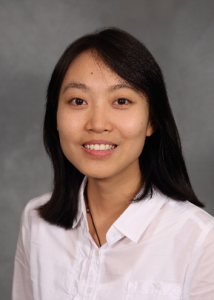
Speaker 2: Miaolan Xie
PhD Candidate in Operations Research and Information Engineering
Cornell University
USA
Abstract:
To handle real-world data that is noisy, biased and even corrupted, we consider a simple adaptive framework for stochastic optimization where the step size is adaptively adjusted according to the algorithm’s progress instead of manual tuning or using a pre-specified sequence. Function value, gradient and possibly Hessian estimates are provided by probabilistic oracles and can be biased and arbitrarily corrupted, capturing multiple settings including expected loss minimization in machine learning, zeroth-order and low-precision optimization. This framework is very general and encompasses stochastic variants of line search, quasi-Newton, cubic regularized Newton and SQP methods for unconstrained and constrained problems. Under reasonable conditions on the oracles, we show high probability bounds on the sample (and iteration) complexity of the algorithms.
Frontier Challenges in AI: The case of algorithmic bias in forecasting tools
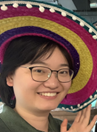
Abstract: In machine learning, training data often capture the behaviour of multiple subgroups of some underlying human population. This behaviour can often be modelled as observations of an unknown dynamical system with an unobserved state. When the training data for the subgroups are not controlled carefully, however, under-representation bias arises. To counter under-representation bias, we introduce two natural notions of fairness in time-series forecasting problems: subgroup fairness and instantaneous fairness. These notions extend predictive parity to the learning of dynamical systems. We also show globally convergent methods for the fairness-constrained learning problems using hierarchies of convexifications of non-commutative polynomial optimisation problems. We also show that by exploiting sparsity in the convexifications, we can reduce the run time of our methods considerably. Our empirical results on a biased data set motivated by insurance applications and the well-known COMPAS data set demonstrate the efficacy of our methods.
October 23, 2023, 16.30 – 17.30 (CET)
Metrizing Fairness
To attend the seminar, click here.
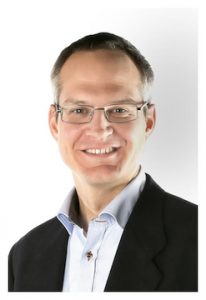
Speaker: Prof Daniel Kuhn
Professor of Operations Research
Risk Analytics and Optimization Chair
College of Management of Technology
EPFL
Switzerland
Abstract: The last decade has witnessed a surge of algorithms that have a consequential impact on our daily lives. Machine learning methods are increasingly used, for example, to decide whom to grant or deny loans, college admission, bail or parole. Even though it would be natural to expect that algorithms are free of prejudice, it turns out that cutting-edge AI techniques can learn or even amplify human biases and may thus be far from fair. Accordingly, a key challenge in automated decision-making is to ensure that individuals of different demographic groups have equal chances of securing beneficial outcomes. In this talk we first highlight the difficulties of defining fairness criteria, and we show that a naive use of popular fairness constraints can have undesired consequences. We then characterize situations in which fairness constraints or unfairness penalties have a regularizing effect and may thus improve out-of-sample performance. We also identify a class of unfairness-measures that are susceptible to efficient stochastic gradient descent algorithms, and we propose a statistical hypothesis test for fairness.
October 30, 2023, 16.30 – 17.30 (CET)
Challenges of Combining Forecasts from Correlated Sources
To attend the seminar, click here.
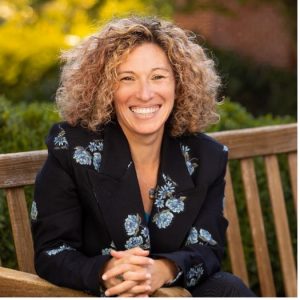
Speaker: Prof Yael Grushka-Cockayne
President Decision Analysis Society, INFORMS
Altec Styslinger Foundation Bicentennial Professor of Business Administration
Senior Associate Dean for Professional Degree Programs
Darden School of Business
University of Virginia
USA
Abstract:
In this talk, we will explore some challenges with forming a consensus forecast when combining forecasts from multiple sources. We will propose the use of a common correlation heuristic for aggregating point forecasts. The forecast aggregation literature has a long history of accounting for correlation among forecast errors. Theoretically sound methods, however, such as covariance-based weights, have been outperformed empirically in many studies by a simple average or weights that account for forecast error variance but assume no correlation. We offer a heuristic that utilizes a common correlation between the forecasters, reducing the number of parameters to be estimated while still accounting for some level of correlation.
November 6, 2023, 16.30 – 17.30 (CET)
Smart Predict-then Optimize for Contextual Linear Optimization: Theory and Computation
To attend the seminar, click here.
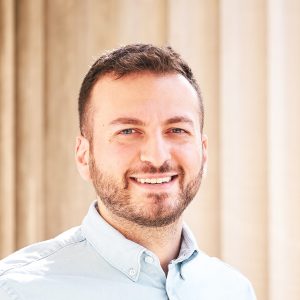
Speaker: Prof Adam Elmachtoub
Associate Professor
Dept. of Industrial Engineering and Operations Research
Columbia University
USA
Abstract:
A standard problem for any analytics researcher or practitioner is to first predict unknown parameters using a machine learning model, and then plug in those parameters into a downstream optimization problem to make a decision. We coin this commonly used practice as predict-then-optimize. In this talk, we discuss a general framework called Smart Predict-then-Optimize (SPO) that integrates the machine learning and optimization components for the setting of contextual linear optimization. We show that our SPO framework lends itself to computationally tractable formulations for training machine learning models that yield improved decisions over natural benchmarks. We also provide theoretical guarantees on consistency and generalization to help explain the performance. This is joint work with Paul Grigas, Ambuj Tewari, Ryan McNellis, Jason Liang, and Othman El Balghiti.
November 13, 2023, 16.30 – 17.30 (CET)
The Minimum Sum-of-Squares Clustering Problem: Robustification and Global Optimization Techniques
To attend the seminar, click here.
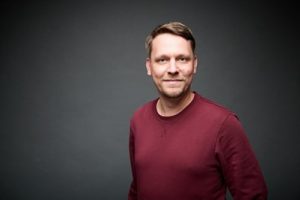
Speaker: Prof Martin Schmidt
Full professor for Nonlinear Optimization
Department of Mathematics
Trier University
Germany
Abstract:
The minimum sum-of-squares clustering (MSSC) problem is an important problem in data mining and (unsupervised) machine learning with very many applications in, e.g., medicine or social sciences. However, it is known to be NP-hard in all relevant cases and to be notoriously hard to be solved to global optimality in practice. Moreover, in many modern applications the clustering suffers from unstructured measurement errors because the MSSC result then represents a clustering of the erroneous measurements instead of the true but unknown underlying data.
In this talk, we discuss both mentioned issues. First, we present different tailored mixed-integer programming techniques to improve the performance of state-of-the-art MINLP solvers when applied to the problem – among them are cutting planes, propagation techniques, branching rules, or primal heuristics. Our numerical study shows that our techniques significantly improve the performance of the open-source MINLP solver SCIP. Second, we tackle the other issue by applying techniques from robust optimization to hedge the clustering result against unstructured errors in the observed data. To this end, we derive strictly and Gamma-robust counterparts. Since the nominal problem is already NP-hard, global approaches are often not feasible in practice. As a remedy, we develop tailored alternating direction methods by decomposing the search space of the robustified problems to quickly obtain feasible points of good quality. Our numerical results reveal an interesting feature: the less conservative Gamma-approach is clearly outperformed by the strictly robust clustering method. In particular, the strictly robustified clustering method is able to recover clusterings of the original data even if only erroneous measurements are observed.
November 20, 2023, 16.30 – 17.30 (CET)
Scientific challenges, practical methodologies and policy perspectives for trustworthy AI
To attend the seminar, click here.
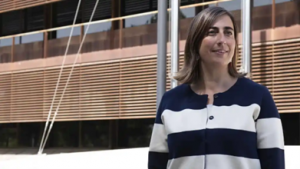
Speaker: Dr Emilia Gomez
Senior Researcher, Joint Research Centre, European Commission, Seville, Spain
Guest Professor of the Department of Information and Communication Technologies, Universitat Pompeu Fabra, Barcelona, Spain
Abstract:
This talk addresses the links between academic research, engineering practices and policy perspectives in the field of trustworthy artificial intelligence (AI) from a European perspective. We provide a summary of the European approach for AI, analyze relevant ethical guidelines and ongoing legal initiatives, and outline some interdisciplinary scientific challenges for ensuring trustworthiness in different types of AI systems, from autonomous driving to music recommender systems.
November 27, 2023, 16.30 – 17.30 (CET)
An interior-point solver for large structured optimization and (data science and alternative) applications
To attend the seminar, click here.
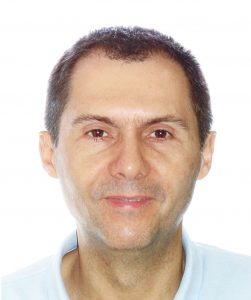
Speaker: Prof Jordi Castro
Professor of Operations Research
Department of Statistics and Operations Research
Universitat Politecnica de Catalunya
Spain
Abstract:
Interior point methods (IPMs) have shown to behave very well in some classes of large structured optimization problems. We will discuss a successful approach for block-angular structures that relies on the efficient solution of the Newton direction. In the first part of the talk we will outline such specialized IPM, which is implemented in a solver named BlockIP. In the second part of the talk we will overview a few (data science an alternative) applications where this algorithm outperformed some of the state-of-the-art solvers, namely: (1) support vector machines; (2) statistical tabular data confidentiality; (3) multistatge stochastic optimization; (4) minimum convex cost flows in bipartite networks.
December 4, 2023, 16.30 – 17.30 (CET)
Adaptive Transport Systems through Operations Research, Behavioral Modeling and Machine Learning
To attend the seminar, click here.
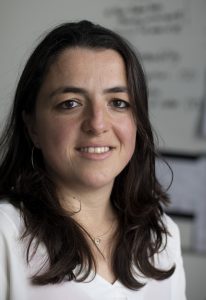
Speaker: Prof Bilge Atasoy
Department of Maritime and Transport Technology
Delft University of Technology
The Netherlands
Talks of Season V
February 6, 2023, 16.30 – 17.30 (CET)
Consistent Second-Order Conic Integer Programming for Learning Bayesian Networks
To attend the seminar, click here.
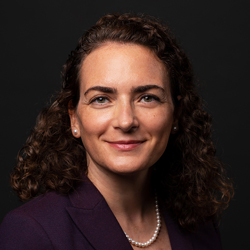
Speaker: Prof Simge Kucukyavuz
Industrial Engineering and Management Sciences Department
Northwestern University
USA
Abstract: Bayesian Networks (BNs) represent conditional probability relations among a set of random variables (nodes) in the form of a directed acyclic graph (DAG), and have found diverse applications in knowledge discovery. We study the problem of learning the sparse DAG structure of a BN from continuous observational data. The central problem can be modeled as a mixed-integer program with an objective function composed of a convex quadratic loss function and a regularization penalty subject to linear constraints. The optimal solution to this mathematical program is known to have desirable statistical properties under certain conditions. However, the state-of-the-art optimization solvers are not able to obtain provably optimal solutions to the existing mathematical formulations for medium-size problems within reasonable computational times. To address this difficulty, we tackle the problem from both computational and statistical perspectives. On the one hand, we propose a concrete early stopping criterion to terminate the branch-and-bound process in order to obtain a near-optimal solution to the mixed-integer program, and establish the consistency of this approximate solution. On the other hand, we improve the existing formulations by replacing the linear big-M constraints that represent the relationship between the continuous and binary indicator variables with second-order conic constraints. Our numerical results demonstrate the effectiveness of the proposed approaches.
February 13, 2023, 16.30 – 17.30 (CET)
Implicit regularization and first order optimization methods
To attend the seminar, click here.
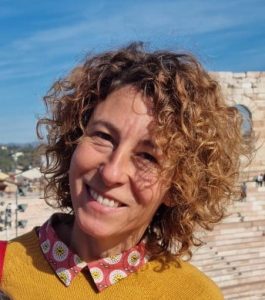
Abstract: First order optimization methods are the algorithms of choice for modern machine earning and inverse problems. In this talk, I will show that the trajectories generated by the chosen algorithm, if suitably initialized, converges to some properly defined minimal “norm» solution. I will discuss the advantages of such approaches with respect to explicit (Tykhonov) regularization strategies and some numerical examples.
February 20, 2023, 16.30 – 17.30 (CET)
AlphaTensor: Discovering faster matrix multiplication algorithms with reinforcement learning
To attend the seminar, click here.
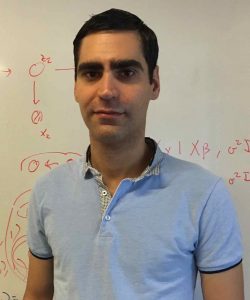
Abstract: Algorithms are the building blocks of computation, and their efficiency in solving fundamental computational tasks is crucial. Matrix multiplication is one such primitive task, occurring in many systems – from machine learning to scientific computing routines. Despite decades of research, the question of how efficiently we can multiply n x m by m x p matrices remains open. Here we develop a Deep Reinforcement Learning approach for discovering efficient and provably correct matrix multiplication algorithms. Our agent, AlphaTensor, is trained to play a single-player tensor decomposition game with a finite factor space, and yields algorithms for multiplying arbitrary matrices. AlphaTensor discovered new algorithms that outperform the state-of-the-art complexity for many matrix sizes (n, m, p). In particular, AlphaTensor improved on Strassen’s two-level algorithm for 4 x 4 matrices in a finite field, which represents the first improvement since its discovery 50 years ago. We further demonstrate the flexibility of AlphaTensor through different use-cases: it discovered novel algorithms with state-of-the-art complexity for structured matrix multiplication, and improved the practical efficiency of matrix multiplication by optimizing for runtime on specific hardware. Our results highlight AlphaTensor’s ability to accelerate the process of algorithmic discovery on a range of problems, and optimizing for different criteria.
February 27, 2023, 16.30 – 17.30 (CET)
Selling personalized upgraded substitutes and co-purchases in online grocery retail
To attend the seminar, click here.
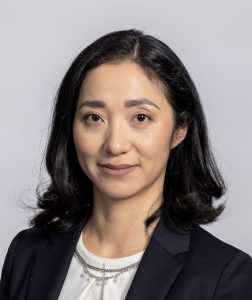
Abstract: In this paper, we investigate how an online grocery retailer can make personalized recommendations for upgraded substitutes and co-purchases as a customer adds items to their shopping basket. Of particular interest is balancing revenue maximization with long-term customer loyalty. We explore three different objectives to model this problem: (i) pure expected revenue maximization, (ii) maximization of a weighted average of the expected revenue and consumer surplus, and (iii) expected revenue maximization with a constraint on the variety of items in a customer’s shopping basket, which is a measure of how much the customer relies on the retailer to meet their needs. We devise fast and efficient algorithms to solve the resulting optimization models and test them out on a large-scale data set from an online grocery retailer. This is joint work with M. Hichame Benbitour (EM Normandie) and Boxiao Chen (UIC).
March 6, 2023, 16.30 – 17.30 (CET)
Submodular maximization of concave utility functions composed with a set-union operator
To attend the seminar, click here.
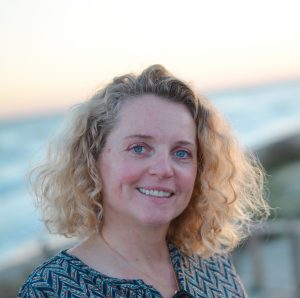
Speaker: Prof Ivana Ljubic
Department of Information Systems, Decision Sciences and Statistics
ESSEC Business School of Paris
France
Abstract: Many important applications in machine learning deal with submodular maximization. Indeed, finding a subset of data points to train neural networks, determining which data points to label to train a classifier, identifying the most influential nodes in a social network, or generating a representative set of diverse recommendations, are some examples of problems that can be solved using techniques of submodular maximization.
In this talk we focus on a family of discrete optimization problems asking for the maximization of the expected value of a concave, strictly increasing, and differentiable function composed with a set-union operator. The expected value is computed with respect to a set of coefficients taking values from a discrete set of scenarios. The function models the utility function of the decision maker, while the set-union operator models a covering relationship between two ground sets, a set of items and a set of metaitems. The goal is to find a subset of metaitems (satisfying certain budget constraints) that maximizes the total utility corresponding to the items it covers. It has applications to, among others, maximal covering location, and influence maximization problems.
The problem can be modeled as a mixed integer nonlinear program (MINLP) involving binary decision variables associated with the items and metaitems. We propose a double-hypograph decomposition which allows for projecting out the variables associated with the items by separately exploiting the structural properties of the utility function and of the set-union operator. Thanks to it, the utility function is linearized via an exact outer-approximation technique, whereas the set-union operator is linearized in two ways: either (i) via a reformulation based on submodular cuts, or (ii) via a Benders decomposition. We analyze from a theoretical perspective the strength of the inequalities of the resulting reformulations, and embed them into two branch-and-cut algorithms. The computational results reveal that, on our testbed of instances, the method based on combining an outer approximation with Benders cuts significantly outperforms the other alternatives.
The talk is based on the article:
- Coniglio, F. Furini, I. Ljubic. Submodular maximization of concave utility functions composed with a set-union operator with applications to maximal covering location problems. Mathematical Programming, 196(1), 9-56 (2022), 2022
March 13, 2023, 16.30 – 17.30 (CET)
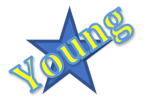
To attend the seminars, click here.
Explainable Data-Driven Optimization: From Context to Decision and Back Again
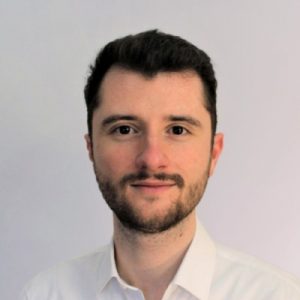
Abstract: Data-driven optimization uses contextual information and machine learning algorithms to find solutions to decision problems with uncertain parameters. While a vast body of work is dedicated to interpreting machine learning models in the classification setting, explaining decision pipelines involving learning algorithms remains unaddressed. This lack of interpretability can block the adoption of data-driven solutions as practitioners may not understand or trust the recommended decisions. We bridge this gap by introducing a counterfactual explanation methodology tailored to explain solutions to data-driven problems. We introduce two classes of explanations and develop methods to find nearest explanations of random forest and nearest-neighbor predictors. We demonstrate our approach by explaining key problems in operations management such as inventory management and routing.
Empowering the User: Finding Regions of Counterfactual Explanations via Robust Optimization
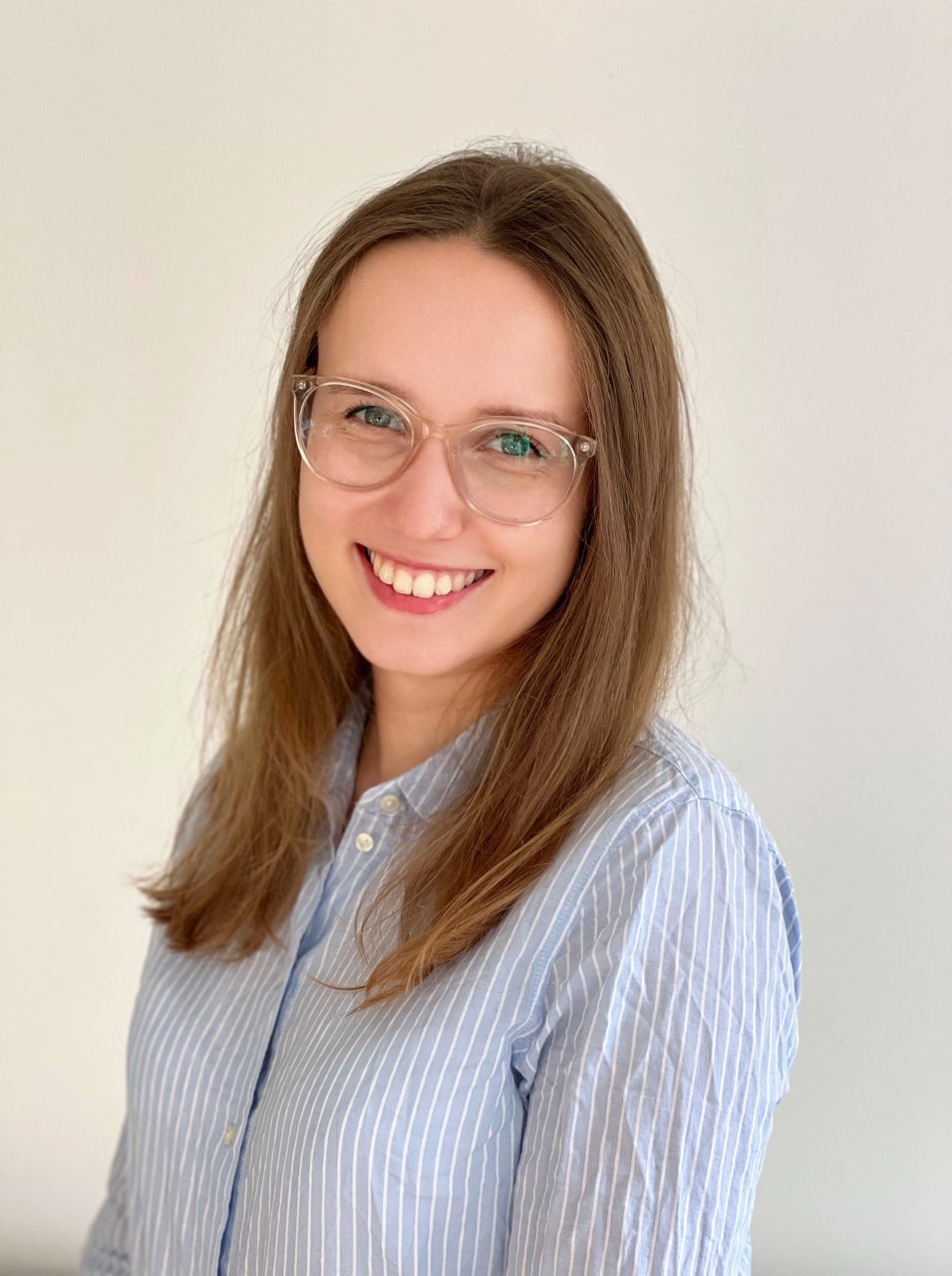
Abstract: Counterfactual explanations play an important role in detecting bias and improving the explainability of data-driven classification models. A counterfactual explanation (CE) is a minimal perturbed data point for which the decision of the model changes. Most of the existing methods can only provide one CE, which may not be achievable for the user. In this work we derive an iterative method to calculate robust CEs, i.e. CEs that remain valid even after the features are slightly perturbed. To this end, our method provides a whole region of CEs allowing the user to choose a suitable recourse to obtain a desired outcome. We use algorithmic ideas from robust optimization and prove convergence results for the most common machine learning methods including logistic regression, decision trees, random forests, and neural networks. Our experiments show that our method can efficiently generate globally optimal robust CEs for a variety of common data sets and classification models.
Revisiting local branching with a machine learning lens
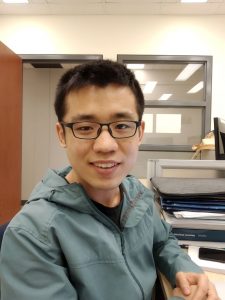
Abstract: Finding high-quality solutions to mixed-integer linear programming problems (MILPs) is of great importance for many practical applications. In this respect, the refinement heuristic local branching (LB) has been proposed to produce improving solutions and has been highly influential for the development of local search methods in MILP. The algorithm iteratively explores a sequence of solution neighborhoods defined by the so-called local branching constraint, namely, a linear inequality limiting the distance from a reference solution. For a LB algorithm, the choice of the neighborhood size is critical to performance. In this work, we study the relation between the size of the search neighborhood and the behavior of the underlying LB algorithm, and we devise a learning based framework for predicting the best size for the specific instance to be solved. Furthermore, we have also investigated the relation between the time limit for exploring the LB neighborhood and the actual performance of LB scheme, and devised a strategy for adapting the time limit. We computationally show that the neighborhood size and time limit can indeed be learned, leading to improved performance and that the overall algorithm generalizes well both with respect to the instance size and, remarkably, across instances.
March 20, 2023, 16.30 – 17.30 (CET)
Applications of Interior Point methods: from Sparse Approximations to Discrete Optimal Transport
To attend the seminar, click here.
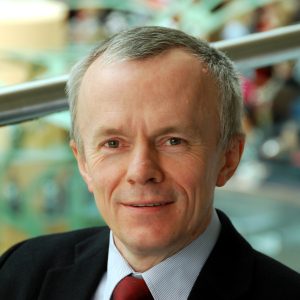
Abstract: A variety of problems in modern applications of optimization require a selection of a ‘sparse’ solution, a vector with preferably few nonzero entries. Such problems may originate from very different applications in computational statistics, signal or image processing or compressed sensing, finance, machine learning and discrete optimal transport, to mention just a few. Sparse approximation problems are often solved with dedicated and highly specialised first-order methods of optimization. In this talk I will argue that these problems may be very efficiently solved by the more reliable optimization techniques which involve some use of the (inexact) second-order information as long as this is combined with appropriately chosen iterative techniques of linear algebra, such as for example methods from the Krylov-subspace family. Two particular classes of methods, the Newton Conjugate Gradient and the Interior Point Method will be interpreted as suitable homotopy type approaches and will be applied to solve problems arising from: compressed sensing, multi-period portfolio optimization, classification of data coming from functional Magnetic Resonance Imaging, restoration of images corrupted by Poisson noise, classification via regularized logistic regression, and discrete optimal transport. In all these cases, the performance of the proposed methods will be compared against competitive first-order methods. Computational evidence will be provided to demonstrate that specialized second-order methods compare favourably and often outperform the cutting-edge first-order methods.
For more details, see:
[1] V. De Simone, D. di Serafino, J. Gondzio, S. Pougkakiotis, and M. Viola, Sparse approximations with interior point methods, SIAM Review 64 (2022) No 4, pp. 954–988. (https://arxiv.org/abs/2102.13608)
[2] F. Zanetti and J. Gondzio, A sparse interior point method for linear programs arising in discrete optimal transport, Tech Report (22 June 2022, revised 6 December 2022). (https://arxiv.org/abs/2206.11009)
March 27, 2023, 16.30 – 17.30 (CET)
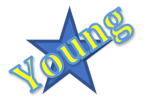
To attend the seminars, click here.
Data-driven Warm-start for Accelerating Short-term Scheduling
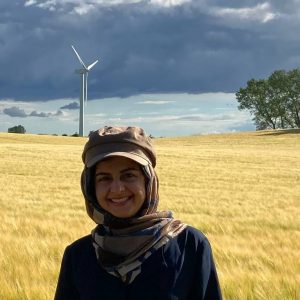
Speaker 1: Dr Farzaneh Pourahmadi
Postdoctoral Researcher
Technical University of Denmark (DTU)
Denmark
Abstract: Most short-term scheduling problems in power systems must be handled frequently by system, market, and transmission operators. However, solving scheduling problems for realistically sized systems can be computationally difficult from optimization perspective, owing to the combination of complex physical laws, binary nature of some decisions and tight latency requirements. This computational complexity is getting worse as more renewables are integrated into the system, as this necessitates resolving complex models at much faster paces unreachable by standard optimization solvers, resulting in a solution with a high optimality gap. In this talk, we describe how we may utilize machine learning to address scheduling problems in a computationally efficient way by predicting binary variables and then using that prediction as an input to solvers. We show how increases in computing efficiency may lead to improved modeling of various types of constraints as well as cost savings.
Learning for Spatial Branching: An Algorithm Selection Approach
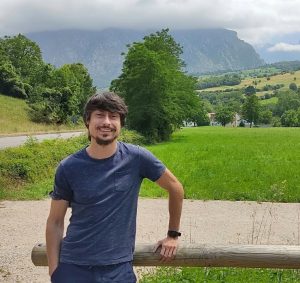
Abstract: The use of machine learning techniques to improve the performance of branch-and-bound optimization algorithms is a very active area in the context of mixed integer linear problems, but little has been done for non-linear optimization. To bridge this gap, we develop a learning framework for spatial branching and show its efficacy in the context of the Reformulation-Linearization Technique for polynomial optimization problems. The proposed learning is performed offline, based on instance-specific features and with no computational overhead when solving new instances. Novel graph-based features are introduced, which turn out to play an important role for the learning. Experiments on different benchmark instances from the literature show that the learning-based branching rule significantly outperforms the standard rules.
FasterRisk: Fast and Accurate Interpretable Risk Scores
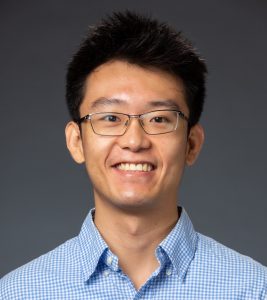
Abstract: Over the last century, risk scores have been the most popular form of predictive model used in healthcare and criminal justice. Risk scores are sparse linear models with integer coefficients; often these models can be memorized or placed on an index card. Typically, risk scores have been created either without data or by rounding logistic regression coefficients, but these methods do not reliably produce high-quality risk scores. Recent work used mathematical programming, which is computationally slow. We introduce an approach for efficiently producing a collection of high-quality risk scores learned from data. Specifically, our approach produces a pool of almost-optimal sparse continuous solutions, each with a different support set, using a beam-search algorithm. Each of these continuous solutions is transformed into a separate risk score through a «star ray» search, where a range of multipliers are considered before rounding the coefficients sequentially to maintain low logistic loss. Our algorithm returns all of these high-quality risk scores for the user to consider. This method completes within minutes and can be valuable in a broad variety of applications.
April 17, 2023, 16.30 – 17.30 (CET)
Tactical Planning under Imperfect Information: A Fast Matheuristic for Two-Stage Stochastic Programs Through Supervised Learning
To attend the seminar, click here.
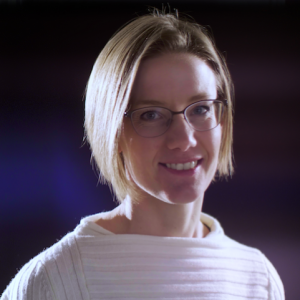
Speaker: Prof Emma Frejinger
Canada Research Chair and holder of the CN Chair in Optimization of Railway Operations
Department of Computer Science and Operations Research
Université de Montréal
Canada
Abstract: In practice, it is often essential to take operational decisions and constraints into account when solving tactical decision-making problems. However, at the tactical level, there is imperfect information about the operational decision-making problems. Transport applications are a rich source of such tactical problems, and they are typically of large scale with difficult operational decision-making problems. In this talk we will use railway transport planning as an illustration.
We propose a methodology at the nexus of operations research and machine learning (ML) leveraging generic approximators available from ML to accelerate the solution of mixed-integer linear two-stage stochastic programs. We aim at solving problems where the second stage is highly demanding. Our core idea is to gain large reductions in online solution time while incurring small reductions in first-stage solution accuracy by substituting the exact second-stage solutions with fast, yet accurate supervised ML predictions. This upfront investment in ML would be justified when similar problems are solved repeatedly over time, for example, in transport planning related to fleet management, routing and container yard management. The proposed method can solve the hardest benchmark instances, on average, in 9-20% of the time it takes the state-of-the-art exact method. Average optimality gaps are in most cases less than 0.1%.
The talk is based on the two following papers:
Larsen, Lachapelle, Bengio, Frejinger, Lacoste Julien, Lodi, Predicting Tactical Solutions to Operational Planning Problems Under Imperfect Information, INFORMS Journal on Computing 34(1):227-242, 2022.
Larsen, Frejinger, Gendron, Lodi, Fast Continuous and Integer L-Shaped Heuristics Through Supervised Learning, arXiv:2205.00897v2, 2022.
April 24, 2023, 16.30 – 17.30 (CET)
Bridging Matching, Regression, and Weighting as Mathematical Programs for Causal Inference
To attend the seminar, click here.
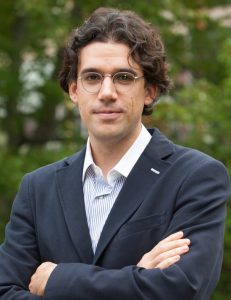
Speaker: Prof José Ramón Zubizarreta
Department of Health Care Policy, Harvard Medical School
Department of Biostatistics, Harvard T.H. Chan School of Public Health
Faculty Affiliate, Department of Statistics, Faculty of Arts and Sciences
Harvard University
USA
Abstract: A fundamental principle in the design of observational studies is to approximate the randomized experiment that would have been conducted under controlled circumstances. Across the health and social sciences, statistical methods for covariate adjustment are used in pursuit of this principle. Basic methods are matching, regression, and weighting. In this talk, we will examine the connections between these methods through their underlying mathematical programs. We will study their strengths and weaknesses in terms of study design, computational tractability, and statistical efficiency. Overall, we will discuss the role of mathematical optimization for the design and analysis of studies of causal effects.
Talks of Season IV
September 19, 2022, 16.30 – 17.30 (CET)
Empirical model learning: machine learning meets optimization
To attend the seminar, click here.
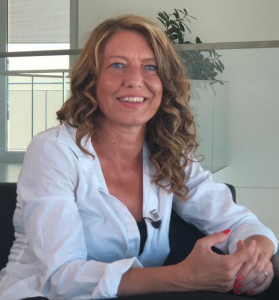
Speaker: Prof Michela Milano
Department of Computer Science and Engineering
Università di Bologna
Italy
Abstract: Designing good models is one of the main challenges for obtaining realistic and useful decision support and optimization systems. Traditionally combinatorial models are crafted by interacting with domain experts with limited accuracy guarantees. Nowadays we have access to data sets of unprecedented scale and accuracy about the systems we are deciding on.
In this talk we propose a methodology called Empirical Model Learning that uses machine learning to extract data-driven decision model components and integrates them into an expert-designed decision model. We outline the main domains where EML could be useful and we show how to ground Empirical Model Learning on a problem of thermal-aware workload allocation and scheduling on a multi-core platform.
In addition, we discuss how to use EML with different optimization and machine learning techniques, and we provide some hints about recent work on EML for hierarchical optimization and on-line/off-line optimization.
September 26, 2022, 16.30 – 17.30 (CET)
Beyond null hypothesis statistical testing: A Bayesian approach to analyze experimental ranking data
To attend the seminar, click here.
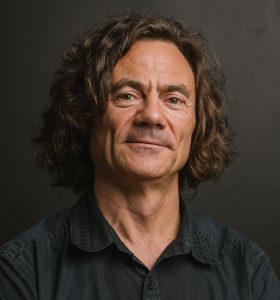
Abstract: In this talk we initially analyze null hypothesis statistical testing, the use of p-values and the controversy around them. Departing from their weaknesses and missuses we provide an alternative based on a Bayesian approach. Particularly we propose a Bayesian method to deal with ranking data generated from machine learning or optimization experiments. The proposed method provides much richer information than that produced for statatistical testing such as quantifying the uncertainty in the comparison. This allows to take much more informative decisions and to carry out deeper analysis. While we illustate the methodology by means of data coming from the results of optimization algorithms, data from classifiers or other machine learning algorithms are also susceptible of being analyzed.
October 3, 2022, 16.30 – 17.30 (CET)
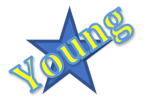
To attend the seminars, click here.
Maximum Margin Optimal Classification Trees
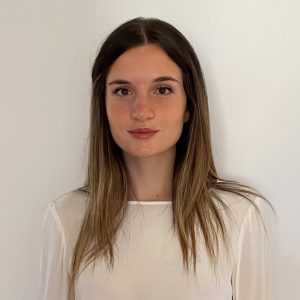
Speaker 1: Marta Monaci
PhD Student
Department of Computer, Control and Management Engineering
Sapienza University of Rome
Italy
Abstract: In recent years there has been growing attention to interpretable machine learning models which can give explanatory insights on their behavior. Thanks to their interpretability, decision trees have been intensively studied for classification tasks, and due to the remarkable advances in mixed-integer programming (MIP), various approaches have been proposed to formulate the problem of training an Optimal Classification Tree (OCT) as a MIP model. We present a novel mixed-integer quadratic formulation for the OCT problem, which exploits the generalization capabilities of Support Vector Machines for binary classification. Our model, denoted as Maximum Margin Optimal Classification Tree (MARGOT), encompasses the use of maximum margin multivariate hyperplanes nested in a binary tree structure. To enhance the interpretability of our approach, we analyse two alternative versions of MARGOT, which include feature selection constraints inducing local sparsity of the hyperplanes. First, MARGOT has been tested on non-linearly separable synthetic datasets in 2-dimensional feature space to provide a graphical representation of the maximum margin approach. Finally, the proposed models have been tested on benchmark datasets from the UCI repository. The MARGOT formulation turns out to be easier to solve than other OCT approaches, and the generated tree better generalizes on new observations.The two interpretable versions are effective in selecting the most relevant features and maintaining good prediction quality.
Conic formulation of QPCCs applied to truly sparse QPs
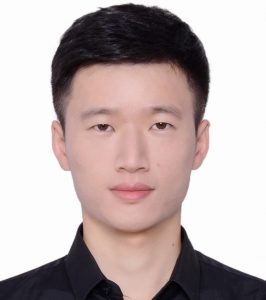
Abstract: We study (nonconvex) quadratic optimization problems with complementarity constraints, establishing an exact completely positive reformulation under — apparently new — mild conditions involving only the constraints, not the objective. Moreover, we also give the conditions for strong conic duality between the obtained completely positive problem and its dual. Another novelty of our approach is a purely continuous model which avoids any branching or use of large constants in implementation. An application to pursuing interpretable sparse solutions of quadratic optimization problems is shown to satisfy our settings, and therefore we link quadratic problems with an exact sparsity term to copositive optimization. The covered problem class includes sparse least-squares regression under linear constraints, for instance. Numerical comparisons between our method and other approximations are reported from the perspective of the objective function value.
Features Compression based on Counterfactual Explanations
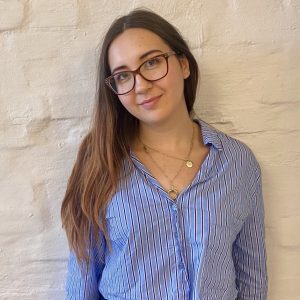
Speaker 3: Cecilia Salvatore
PhD Student
Department of Civil Engineering and Computer Science
University of Rome Tor Vergata
Italy
Abstract: Automated decision-making classification systems based on Machine Learning algorithms are often used in many real-life scenarios such as healthcare, credit, or criminal justice. There is thus increasing interest in making Machine Learning systems trustworthy: interpretability, robustness, and fairness are often essential requirements for deploying these systems. In particular, according to the European Union’s General Data Protection Regulation (GDPR), automated decision-making systems should guarantee the »right to explanations», meaning that those affected by the decision may require an explanation. Counterfactual Explanations are becoming a de-facto standard for a post-hoc explanation. Given an instance of a classification problem belonging to a class, its counterfactual explanation corresponds to small perturbations of that instance that allow changing the classification outcome. This work aims to leverage Counterfactual Explanations to detect the important decision boundaries of a pre-trained black-box model and to use this information to select and discretize the dataset’s features with a tunable granularity. This information can then be used to train a small and interpretable Decision Tree that will be more robust and not prone to overfitting.
October 10, 2022, 16.30 – 17.30 (CET)
Scaling Optimal Transport for High dimensional Learning
To attend the seminar, click here.
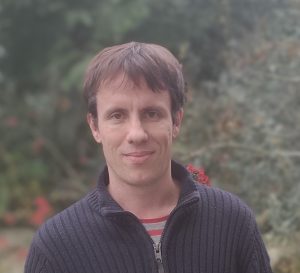
Abstract: Optimal transport (OT) has recently gained a lot of interest in machine learning. It is a natural tool to compare in a geometrically faithful way probability distributions. It finds applications in both supervised learning (using geometric loss functions) and unsupervised learning (to perform generative model fitting). OT is however plagued by the curse of dimensionality, since it might require a number of samples which grows exponentially with the dimension. In this talk, I will explain how to leverage entropic regularization methods to define computationally efficient loss functions, approximating OT with a better sample complexity. More information and references can be found on the website of our book «Computational Optimal Transport» https://optimaltransport.github.io/
.October 24, 2022, 16.30 – 17.30 (CET)
Clusters everywhere: Clustering methods for Global Optimization, Global Optimization for Clustering, with application to atomic Clusters
To attend the seminar, click here.
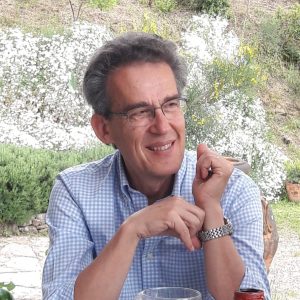
Abstract: In this talk I will start by showing how it is possible to use clustering methods to improve some Global Optimization (GO) methods; this idea was first proposed in the very beginning of GO in the 70’s, but later it was abandoned. We re-discovered this methodology in recent years and produced variants which, for some problem classes, are quite promising. One of such classes is that of detecting the minimum energy configuration of clusters of atoms.
In the second part of the talk, I will consider clustering as a hard to solve GO optimization problem itself and will outline some ideas on how to modify some standard GO heuristics in order to make clustering more efficient.
October 31, 2022, 16.30 – 17.30 (CET)
AI Explainability and Trust
To attend the seminar, click here.
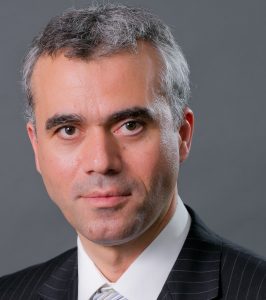
Abstract: Discussions about trustworthy and responsible AI have become central across multiple communities in recent years – machine learning, law, social sciences, among others. A key challenge regarding trust in AI – also considered important by regulators as part of transparency for some AI applications – is to understand why “black boxes” may be making specific predictions. As a result, explainable AI (XAI) has been a growing topic of research. In this talk, I will discuss some potential drawbacks XAI may have – including the potential to erode risk in practice – and also present some work that takes into account behavioral aspects researchers and practitioners may need to consider when developing XAI.
November 7, 2022, 16.30 – 17.30 (CET)
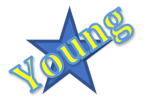
To attend the seminars, click here.
Pruning in Deep Learning by Optimization
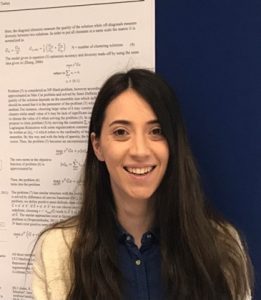
Speaker 1: Buse Cisil Guldogus
PhD Candidate
Department of Industrial Engineering
Bahcesehir University
Turkey
Abstract: Ensemble methods are highly preferred in machine learning since it combines several models to produce an optimal predictive model. Ensemble pruning helps to reduce the computational complexity of ensemble models by selecting a subset of classifiers where accuracy and diversity conflict with each other. The ensemble concept can be used in deep learning models to provide robustness and reliability. Hence, this study focuses on developing a novel mathematical model to prune the ensemble of Convolutional Neural Networks (CNN) with different depths and layers with sparse second order cone programming where accuracy and diversity trade-off is optimized. The proposed model is tested on CIFAR-10, CIFAR-100 and MNIST data set to obtain performance metrics which gives promising results by reducing the number of models significantly.The same proposed model is also applied as an embedded feature selection algorithm by training a neural network on multiple single features of a dataset and using their respective outputs to generate a probability class distribution along with a prediction. This methodology allows implementation of second-order conic programming to determine features that contribute most to accurate classification using neural networks as embedded classifiers.
Credit Limit Adjustment using Reinforcement Learning
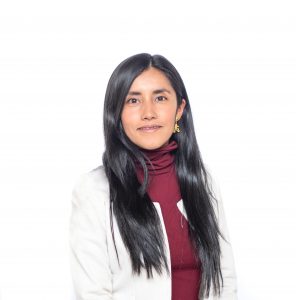
Speaker 2: Sherly Paola Alfonso Sanchez
PhD Student
Department of Statistical and Actuarial Sciences
Western University, Canada
Auxiliary Professor at Universidad Nacional de Colombia
Abstract: Reinforcement learning (RL) has been explored for many problems, from video games with deterministic environments to portfolio and operations management where the scenarios are stochastics. In our work, we seek to find and automatize an optimal policy to credit limit adjustment, a fundamental problem for banking and fintech companies that offer credit card products. This problem requires the optimization of the expected profit, given the performed actions for the portfolio of credit card customers; with this purpose, we have to balance two different objectives: first, maximize the portfolio’s renewal and second, minimize the portfolio’s provisions.
To apply reinforcement learning techniques, we use an offline learning strategy that needs to simulate the actions’ impact based on historical data provided by a Supper-App company in Latin America. Our work is a proposal that employs RL techniques to credit limit adjustment, methodology that until today has not been widely explored. Besides, our research gives insights into the result of alternative data usage to make decisions about adjustment of credit limits in credit card portfolios as well as, a new objective technique to make these decisions primarily based on data-driven methods instead of only relying on expert-driven systems.
Building replicable models for energy grid management: A Graph Approach From RENergetic
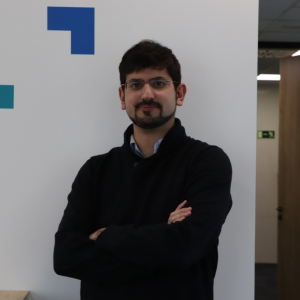
Abstract: The use of renewable energy sources and the optimization of the consumption in smaller communities is becoming more and more important as the challenges of climate change arise. Energy islands seek to achieve the highest possible degree of self-sustainability by means of the optimization in the use of available energy sources. As part of RENErgetic, an EC funded project, we strive to best approach to be able to handle energy data so that energy communities can rapidly have their own management system. In this talk, we will discuss about this topic and the solution developed using graph analysis as a means to represent and handle energy measurements as well as what it entitles for the forecasting effort. With this we intent to provide easy-to-use forecasting models as well as information of the consumption that engages people within the community to work towards this common goal.
November 14, 2022, 16.30 – 17.30 (CET)
Distributed Systems for Decentralized AI: Last Decade and Beyond
To attend the seminar, click here.
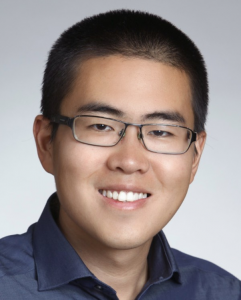
Abstract: Today’s machine learning models are trained dominantly in a centralized environment such as data centers. Such a strong dependency on closely coupled interconnections is causing problems not only with the cost of infrastructure, contributing to the staggering cost of model training (not uncommon to be in the million-dollar regime!) but also with the transparency and openness of today’s machine learning ecosystem. While a decentralized paradigm has been successfully applied to various other areas such as SETI@HOME and Folding@HOME, their adoption to machine learning comes with great challenges — today’s optimization algorithms that are designed for fast networks are inherently communication heavy. To bring AI into a decentralized future, we need to fundamentally rethink the training algorithm, system design, and hardware accelerations, all together.
In this talk, I will provide a personal reflection on our efforts in decentralized learning over the last decade, and put them into the context of many fascinating work that has been conducted by the community during the same period of time. I will also discuss my personal view on the future of decentralized learning and a “cry for help” with many challenges that we are still struggling with.
November 21, 2022, 16.30 – 17.30 (CET)
The Synergy of Data Analytics, Machine Learning and Statistics into Hybrid Models with Healthcare Applications
To attend the seminar, click here.
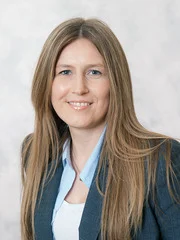
Abstract: In the past decade, there has been an overwhelming acceptance for data analytics, machine learning and statistical modelling with developments in the field starting to gain momentum. More and more industries and public sector bodies are investing in data and data scientists however there is still much work to be done to embed the sophisticated algorithms from research into real world applications. There is also a need for understanding what methods should be used whether that be traditional approaches which can be just as effective in certain cases, new techniques or multiple approaches needed in collaboration.
In this talk, the intention is to describe traditional survival models and how we complement statistical methodologies such as survival modelling and data mining to answer real life challenges, with a view of further developing the work to real-time survival models. This will be used as an example of how hybrid models have developed and can be utilised.
The talk will draw on personal experiences of developing hybrid models and embedding statistical data analytics into everyday applications to inform real-time decision making.
December 5, 2022, 16.30 – 17.30 (CET)
Variational Bayes for high-dimensional linear and logistic regression
To attend the seminar, click here.
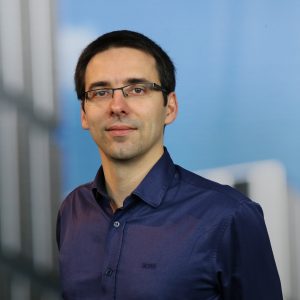
Abstract: Bayesian methods are becoming increasingly popular in high- and infinite dimensional models. However, standard, MCMC based methods are known to scale badly with the sample size and number of parameters in complex models. Therefore, in practice often variational algorithms are used to approximate the posterior distribution. These methods up to recently were black box procedures with very limited theoretical underpinning. In this talk we discuss variational Bayes methods for the high-dimensional linear and logistic regression models, derive theoretical frequentist statistical guarantees for them and investigate algorithmic aspects through numerical analysis. The talk is based on a joint work with Kolyan Ray and Gabriel Clara.
December 12, 2022, 16.30 – 17.30 (CET)
Packing hypertrees and the k-cut problem in Hypergraphs
To attend the seminar, click here.
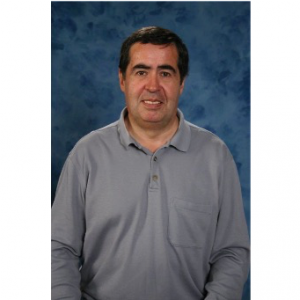
Abstract: We give a combinatorial algorithm to find a maximum packing of hypertrees in a capacitated hypergraph. Based on this we extend to hypergraphs several algorithms for the k-cut problem, that are based on packing spanning trees in a graph. In particular, we give a γ-approximation algorithm for hypergraphs of rank γ, extending the work of Ravi and Sinha [24] for graphs. We also extend the work of Chekuri, Quanrud and Xu [7] in graphs, to give an algorithm for the k-cut problem in hypergraphs that is polynomial if k and the rank of the hypergraph are fixed. We also give a combinatorial algorithm to solve a linear programming relaxation of this problem in hypergraphs.
Talks of Season III
February 7, 2022, 16.30 – 17.30 (CET)
Permutation compressors for provably faster distributed nonconvex optimization
To attend the seminar, click here.
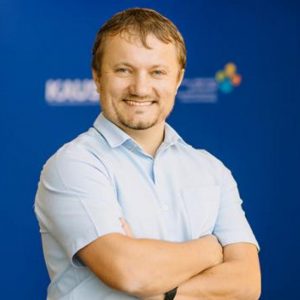
Speaker: Prof Peter Richtarik
Professor of Computer Science
Professor of Applied Mathematics
& Computational Science (by courtesy)
Professor of Statistics (by courtesy)
King Abdullah University of Science and Technology
Kingdom of Saudi Arabia
Abstract: We study the MARINA method of Gorbunov et al (2021) — the current state-of-the-art distributed non-convex optimization method in terms of theoretical communication complexity. Theoretical superiority of this method can be largely attributed to two sources: the use of a carefully engineered biased stochastic gradient estimator, which leads to a reduction in the number of communication rounds, and the reliance on independent stochastic communication compression operators, which leads to a reduction in the number of transmitted bits within each communication round. In this paper we i) extend the theory of MARINA to support a much wider class of potentially correlated compressors, extending the reach of the method beyond the classical independent compressors setting, ii) show that a new quantity, for which we coin the name Hessian variance, allows us to significantly refine the original analysis of MARINA without any additional assumptions, and iii) identify a special class of correlated compressors based on the idea of random permutations, for which we coin the term PermK, the use of which leads to O(√n) (resp. O(1+d/√n)) improvement in the theoretical communication complexity of MARINA in the low Hessian variance regime when d≥n (resp. d≤n), where n is the number of workers and d is the number of parameters describing the model we are learning. We corroborate our theoretical results with carefully engineered synthetic experiments with minimizing the average of nonconvex quadratics, and on autoencoder training with the MNIST dataset.
See paper at https://arxiv.org/abs/2110.03300.
February 14, 2022, 16.30 – 17.30 (CET)
Modeling multivariate time series with Bayesian networks
To attend the seminar, click here.
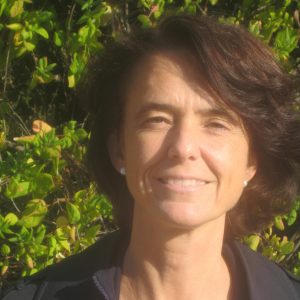
Speaker: Prof Concha Bielza
Department of Artificial Intelligence
Technical University of Madrid
Spain
Abstract: This talk will describe how Bayesian network models can cope with multivariate temporal data. After a brief introduction to Bayesian networks in static domains, discrete-time versions will be presented, with special focus on dynamic Bayesian networks. The more recent continuous time Bayesian networks and their supervised classification counterparts, both in uni- and multi-dimensional settings, will be then explained. Real-world examples from the industry will illustrate all models.
February 21, 2022, 16.30 – 17.30 (CET)
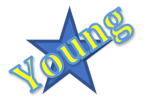
To attend the seminars, click here.
A clustered approach to Data Envelopment Analysis
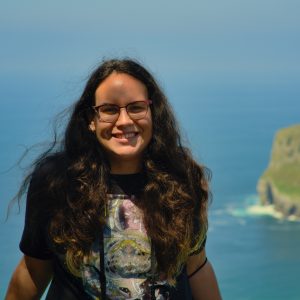
Abstract: In this talk, we tackle the feature selection as well as the peer selection problems in Data Envelopment Analysis jointly. The goal is to cluster the Decision Making Units and develop a more targeted model for each of the clusters to maximize the average efficiency. This is formulated as a Mixed Integer Linear Programming problem and a collection of constructive heuristics is developed. The clustered approach is illustrated on a real-world dataset from the benchmarking of electricity Distribution System Operators, as well as some simulated datasets.
Mixed-Integer Optimization with Constraint Learning
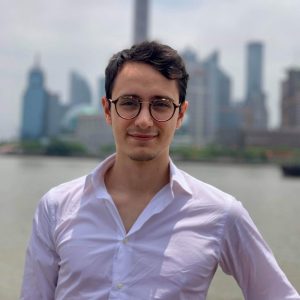
Abstract: We establish a broad methodological foundation for mixed-integer optimization with learned constraints. We propose an end-to-end pipeline for data-driven decision making in which constraints and objectives are directly learned from data using machine learning, and the trained models are embedded in an optimization formulation. We exploit the mixed-integer optimization-representability of many machine learning methods, including linear models, decision trees, ensembles, and multi-layer perceptrons. The consideration of multiple methods allows us to capture various underlying relationships between decisions, contextual variables, and outcomes. We also characterize a decision trust region using the convex hull of the observations, to ensure credible recommendations and avoid extrapolation. We efficiently incorporate this representation using column generation and clustering. In combination with domain-driven constraints and objective terms, the embedded models and trust region define a mixed-integer optimization problem for prescription generation. We implement this framework as a Python package (OptiCL) for practitioners. We demonstrate the method in both chemotherapy optimization and World Food Programme planning. The case studies illustrate the benefit of the framework in generating high-quality prescriptions, the value added by the trust region, the incorporation of multiple machine learning methods, and the inclusion of multiple learned constraints.
Joint work with Holly Wiberg, Dimitris Bertsimas, S. Ilker Birbil, Dick den Hertog, Adejuyigbe Fajemisin.
See paper at https://arxiv.org/abs/2111.04469.
Adaptive Gradient Descent without Descent
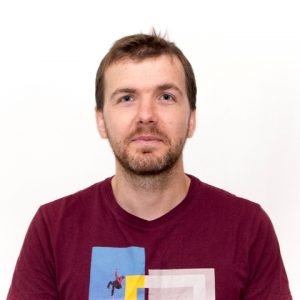
Abstract: In this talk I will present some recent results for the most classical optimization method — gradient descent. We will show that a simple zero cost rule is sufficient to completely automate gradient descent. The method adapts to the local geometry, with convergence guarantees depending only on the smoothness in a neighborhood of a solution. The presentation is based on a joint work with K. Mishchenko.
See paper at https://arxiv.org/abs/1910.09529.
February 28, 2022, 16.30 – 17.30 (CET)
APS based optimization for adversarial machine learning
To attend the seminar, click here.
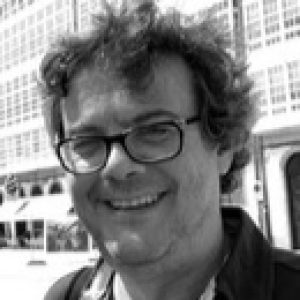
Speaker: Prof David Ríos
AXA-ICMAT Chair in Adversarial Risk Analysis
Member of the Spanish Royal Academy of Sciences
Professor of Statistics and Operations Research
at the Complutense University (on leave)
Spain
Abstract: Adversarial machine learning (AML) aims at protecting machine learning systems from the attacks of adversaries. It is relevant in many areas from security to cybersecurity going through automated driving systems and medicine. The prevalent paradigm in AML has been game theory; I shall sketch an alternative better-founded Bayesian approach to AML. In any case, both lead to tough optimization problems and I shall explain how augmented probability simulation may facilitate a way forward in such context.
Joint work with R. Naveiro, T. Ekin and A. Torres.
March 7, 2022, 16.30 – 17.30 (CET)
Convex hulls of monomials in two-variable cones
To attend the seminar, click here.
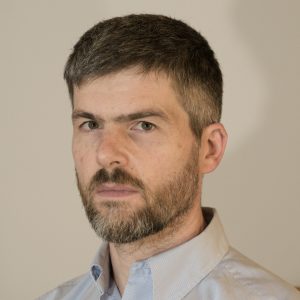
Speaker: Dr Pietro Belotti
Department of Electronics, Information and Bioengineering
Politecnico di Milano
Italy
Abstract: Many exact solvers for discrete nonconvex optimization are based on a reformulation step where expressions are broken into smaller components and a convex relaxation of each component (ideally its convex hull) is sought. These components are uni- or multivariate functions of variables that are restricted to a bounding box, but the functions themselves are sometimes bounded in value. Explicit bounds on a function value can lead to tighter relaxations and hence to better solver performance.
This talk will focus on the convex hull of a monomial function in two variables with real positive exponents, in the special case where the two variables are constrained to a convex cone within the first quadrant rather than a bounding box. Depending on the value of the exponents, the convex hull can be found to have either linear or conic inequalities. I will also share some considerations on the volume of such convex hull and how the volume changes when applying branching rules within branch-and-bound solvers for such problems.
March 14, 2022, 16.30 – 17.30 (CET)
Fairness in Machine Learning: how to quantify the trade-off between accuracy and fairness with an application in structural econometry
To attend the seminar, click here.
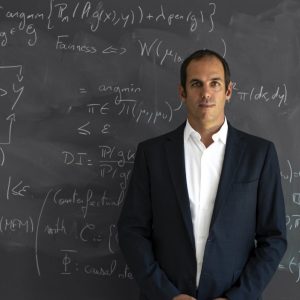
Abstract: A supervised machine learning algorithm builds a model from a learning sample and then use the model to predict new observations. To this end, it aggregates individual characteristics of the observations of the learning sample. But this information aggregation does not consider any potential selection on unobservables and any status-quo biases, which may be contained in the training sample. The latter bias has raised concerns around the so-called fairness of machine learning algorithms, especially towards disadvantaged groups. In this talk we review the issue of fairness in machine learning and study whether it is possible to achieve fairness while conserving accuracy. We provide an example from the study of the instrumental variable regression in structural economy.
March 21, 2022, 16.30 – 17.30 (CET)
Modeling and duality in domain specific languages for mathematical optimization
To attend the seminar, click here.
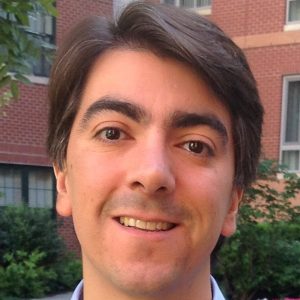
Speaker: Dr Juan Pablo Vielma
Research Scientist at Operations Research Group, Google Research
Research Scientist at Sloan School of Management, MIT
USA
Abstract: Domain specific languages (DSL) for mathematical optimization allow users to write problems in a natural algebraic format. However, what is considered natural can vary from user to user. For instance, JuMP’s interface makes a distinction between conic formulations and nonlinear programming formulations whose constraints are level-sets of nonlinear functions. Tradeoffs between such alternative modeling formats are further amplified when dual solutions are considered. In this talk we describe work related to these tradeoffs in JuMP and OR-Tools. In particular, we consider modeling using a wide range of non-symmetric cones (and their solution with Hypatia.jl) and defining precise dual contracts for two-sided constraints (and other non-conic convex constraints).
March 28, 2022, 16.30 – 17.30 (CET)
The Counterfactual Explanation
To attend the seminar, click here.
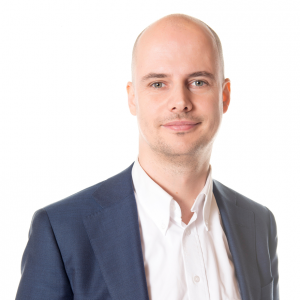
Abstract: The inability of many “black box” prediction models to explain the decisions made, have been widely acknowledged. Explaining the predictions of such models has become an important ethical component and has gained increasing attention of the AI research community, resulting in a new field termed “Explainable AI” (XAI). Counterfactual (CF) explanations has emerged as an important paradigm in this field, and provides evidence on how a (1) prediction model came to a (2) decision for a (3) specific data instance. In this talk, I’ll first provide an introduction to the counterfactual explanation and compare it to other popular approaches. Next, some counterfactual generating techniques are discussed for tabular, textual, behavioral and image data, accompanied with some example applications. Finally, we’ll briefly discuss what lies ahead.
April 4, 2022, 16.30 – 17.30 (CET)
An exact algorithm for the semi-supervised minimum sum of squares clustering
To attend the seminar, click here.
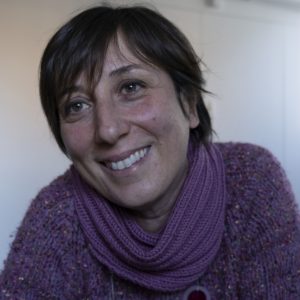
Speaker: Prof Veronica Piccialli
Department of Computer, Control and Management Engineering
Sapienza University of Rome
Italy
Abstract: The minimum sum-of-squares clustering (MSSC), or k-means type clustering, is traditionally considered an unsupervised learning task. In recent years, using background knowledge to improve the cluster quality and promote interpretability of the clustering process has become a hot research topic at the intersection of mathematical optimization and machine learning research. Taking advantage of background information in data clustering leads to the so-called semi-supervised or constrained clustering. In this talk, we consider both instance-level constraints, requiring that pairs of points must or must not be in the same cluster, and cardinality constraints, implying knowledge on the number of elements in each cluster. We define both an exact and a heuristic algorithm that also returns an optimality gap. Both approaches rely on semidefinite programming. Numerical results show that our methods outperform state-of-the-art algorithms, significantly increasing the size of the instances either solved to global optimality or with an optimality gap of less than 1%.
This is a joint work with Antonio Maria Sudoso from Sapienza University of Rome, and Anna Russo Russo for University of Rome Tor Vergata
April 25, 2022, 16.30 – 17.30 (CET)
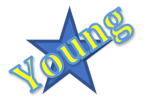
To attend the seminars, click here.
Adversarial attacks against Bayesian forecasting dynamic models
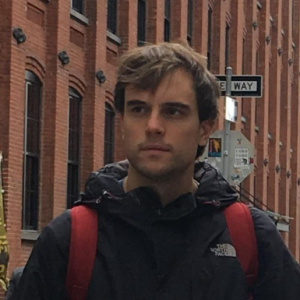
Speaker 1: Dr Roi Naveiro
Postdoctoral researcher
Institute of Mathematical Sciences (ICMAT-CSIC)
Spain
Abstract: The last decade has seen the rise of Adversarial Machine Learning AML). This discipline studies how to manipulate data to fool inference engines, and how to protect those systems against such manipulation attacks. Extensive work on attacks against regression and classification systems is available, while little attention has been paid to attacks against time series forecasting systems. In this paper, we propose a decision analysis based attacking strategy that could be utilized against Bayesian forecasting dynamic models.
See paper at https://arxiv.org/pdf/2110.10783.pdf.
Learning Linear Representations of Nonlinear Dynamics Using Deep Learning
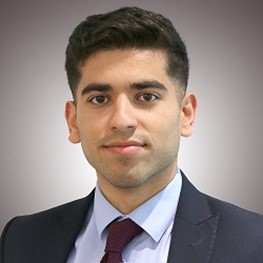
Speaker 2: Akhil Ahmed
PhD student in the Department of Chemical Engineering, Imperial College London.
Member of the Autonomous Industrial Systems Lab (Headed by supervisor Dr Mehmet Mercangoz) and Optimisation and Machine Learning for Process Engineering group (Headed by supervisor Dr Antonio del Rio-Chanona).
Member of The Sargent Centre for Process Systems Engineering
UK
Abstract: The vast majority of systems of practical interest are characterised by nonlinear dynamics. This renders the control and optimization of such systems a complex task due to their nonlinear behaviour. In contrast, the study of linear systems is well developed with scalable control and optimization algorithms thoroughly detailed within the literature. For this reason, we propose a new deep learning framework to discover a transformation of a nonlinear dynamical system to an equivalent higher dimensional linear representation. Within the proposed framework, the synergy between optimization and machine learning is exploited. Specifically, the neural network serves the role of parameterizing the function space over which the transformation is searched for, allowing for a tractable optimization problem to be solved. Ultimately, we demonstrate that the resulting learned linear representation accurately captures the dynamics of the original system for a wide range of conditions. Consequently, we show that the learned linear model can be used for the successful control of the original system. This is demonstrated through a series of case studies.
Exploring study profiles of Computer Science students with Social Network Analysis
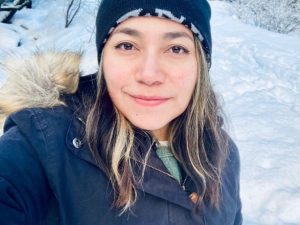
Abstract: Information technology is widely adapted in all levels of education. The extensive information resources facilitate enhanced human capacity and the social environment to support learning. In particular, Social Network Analysis (SNA) has been broadly used in teaching and learning practices. In this talk, we will present concepts of SNA, community detection methods, and the community detection analysis applied to identify the learning behavior profiles of undergraduate computer science students in a Nordic university. We analyze the biggest communities to identify the factors that characterize the students’ learning strategy and preferences.
See paper at:
May 2, 2022, 16.30 – 17.30 (CET)
Machine Learning, Artificial Intelligence and Optimization: Opportunities for Inter-Disciplinary Innovation
To attend the seminar, click here.
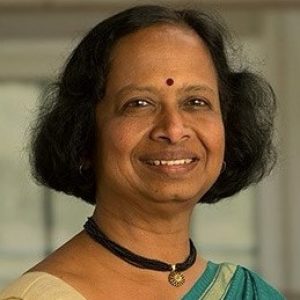
Speaker: Dr Radhika Kulkarni
Vice President (Retired), Advanced Analytics R&D, SAS Institute Inc.
2022 President of INFORMS
USA
Abstract: Machine learning tools and AI platforms have become prolific in many industries. Applications range from health care to financial applications to manufacturing industries. In the world of big data and ML/AI tools, there are numerous opportunities for application of optimization techniques. Large scale implementation of machine learning tools in artificial intelligence platforms require automation at several levels – increasing productivity along the entire analytics lifecycle as well as automated model selection to improve predictive models. In many of these problems, optimization techniques play an important role in finding solutions as well as improving performance.
This presentation will provide several examples that describe some of these innovations in various industries as well as discuss trends and upcoming challenges for future research.
Often, the mathematical model and solution are only a small part of the overall problem. It is also important to ensure the availability of the data required for the model, whether the result is easy to interpret and sustainable in the real world and myriad other aspects. In this talk, Kulkarni will discuss some of the practical concerns that are of equal importance: ease of implementation, acceptance of the results, safeguards needed to allow for over-rides of automatic decisioning, etc.
Talks of Season II
September 20, 2021, 16.30 – 17.30 (CET)
Random Projections in Mathematical Programming
To attend the seminar, click here.
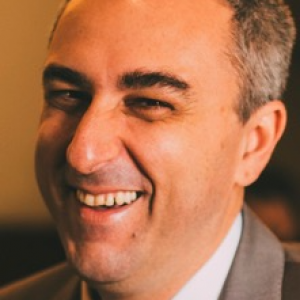
Abstract: We present theoretical and computational results relating to a set of works where we apply random projection techniques to mathematical programming formulation classes, namely linear programs, conic programs, quadratic programs, and one mixed-integer nonlinear program (the k-means problem). Time permitting, we comment on applications of these techniques to the diet problem, quantile regression, compressed sensing, and portfolio optimization.
Joint work with C. D’Ambrosio, B. Manca, P.-L. Poirion, K. Vu
October 4, 2021, 16.30 – 17.30 (CET)
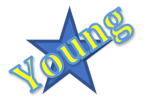
To attend the seminars, click here.
On linear regression models with hierarchical categorical variables
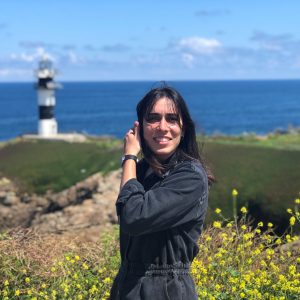
Speaker 1: M. Remedios Sillero-Denamiel
Postdoctoral Fellow
School of Computer Science and Statistics
Trinity College Dublin
Ireland
Abstract: We study linear regression models built on categorical predictor variables that have a hierarchical structure, with their categories arranged as a directed tree. While the categories in the leaf nodes give the highest granularity in the representation of these variables, the user may decide to go upstream the tree and consolidate individuals at ancestor nodes, sharing the same coefficient. This reduced model, with fewer coefficients to be estimated, is easier to interpret, and hopefully does not damage the accuracy. We study the mathematical optimization problem that trades off the accuracy of the reduced linear regression model and its complexity, measured as a cost function of the level of granularity of the representation of the hierarchical categorical variables. We show that finding non-dominated outcomes for this problem boils down to solving a Mixed Integer Convex Quadratic Problem with Linear Constraints. Our experiments show that a much less complex model with a very mild worsening of the accuracy can be obtained.
This is a joint work together with Emilio Carrizosa, Laust Hvas Mortensen and Dolores Romero Morales.
See paper at
On a Mathematical Optimization approach to constrained smoothing and out-of-range prediction
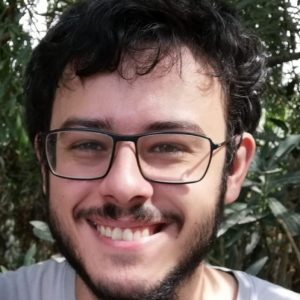
Abstract: Designing systems which incorporate human knowledge and provide valuable support to the decider is crucial to guide decision-making in the context of complex and evolving data. In this work, statistical modelling and mathematical optimization paradigms merge to address the problem of estimating smooth curves in a regression setting which are defined through a reduced-rank basis and verify structural properties, both in the observed domain in which data have been gathered and outwards. If a smooth curve is fitted using an unconstrained approach, misleading results might be depicted, e.g., negative estimated values of the response variable when only positive values are observed. To avoid this and related situations, we propose to embed requirements about their sign, monotonicity and curvature in a penalized splines (P-splines) fitting process as hard constraints, yielding a semidefinite optimization model. Furthermore, making out-of-range predictions under a different set of constrained scenarios, such as having an estimated growth rate, is also possible using the approach developed in this work. The usefulness of our approach is illustrated in both simulated and real data, last arising in a demographic application and the study of the evolution of the COVID-19 pandemic.
See paper at
Learning to Sparsifying Travelling Salesman Problem Instances
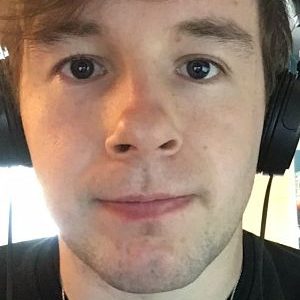
Speaker 3: James Fitzpatrick
PhD student
Quinn School of Business
University College Dublin
Ireland
Abstract: We present our experiments investigating the use of classical machine learning classification methods to develop a pruning heuristic for instances of the classical travelling salesman problem. This is posed as a response to the recent interest in the use of end-to-end deep learning methods for solving NP-hard combinatorial optimisation problems, which have issues of representation, generalisation, complexity, and interpretability. Our technique is introduced as a pre-processing step followed by an exact integer programming approach where, using carefully-selected features derived from cutting planes exploration, minimum spanning tree heuristics and other analysis of the graph edge weights, we learn which edges in the underlying graph are unlikely to belong to an optimal solution. We remove these edges, significantly reducing the number of decision variables in the pruned optimisation problem. This approach can be used for problem instances even if they lie outside the training distribution, resulting in typically small optimality gaps between the pruned and original problems in most cases.
October 11, 2021, 16.30 – 17.30 (CET)
Learning from user and environment in combinatorial optimisation
To attend the seminar, click here.
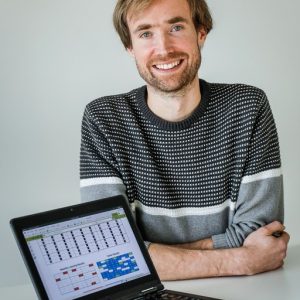
Abstract: Industry and society are increasingly automating processes, which requires solving constrained optimisation problems. This includes vehicle routing problems in transportation, scheduling of tasks in electricity demand-response programs, rostering of personnel and more. However, the solutions found by state-of-the-art constraint programming solvers are often found to not match the expectations of domain experts, which reduces the potential of the technology.
One key direction to overcome this, is to automatically learn from the user and environment over time. This includes learning preferences, implicit constraints and impacts of external factors. It requires combining machine learning techniques with constraint solving techniques, and hence hybrid learning and reasoning systems.
In this talk I will provide an overview of three different ways in which part of the problem specification can be learned from data. This includes preference learning of objective functions, perception-based reasoning and end-to-end decision focussed learning, where I will highlight recent evolutions and advances we have been making in our lab and how they fit in the wider research area.
October 18, 2021, 16.30 – 17.30 (CET)
Optimal counterfactual explanations in tree ensembles
To attend the seminar, click here.
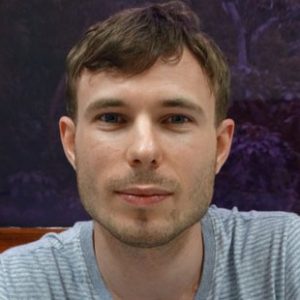
Speaker: Prof Thibaut Vidal
Scale-AI Chair in Data-Driven Supply Chains
MAGI
Polytechnique Montréal
Canada
Abstract: Counterfactual explanations are usually generated through heuristics that are sensitive to the search’s initial conditions. The absence of guarantees of performance and robustness hinders trustworthiness. In this paper, we take a disciplined approach towards counterfactual explanations for tree ensembles. We advocate for a model-based search aiming at «optimal» explanations and propose efficient mixed-integer programming approaches. We show that isolation forests can be modeled within our framework to focus the search on plausible explanations with a low outlier score. We provide comprehensive coverage of additional constraints that model important objectives, heterogeneous data types, structural constraints on the feature space, along with resource and actionability restrictions. Our experimental analyses demonstrate that the proposed search approach requires a computational effort that is orders of magnitude smaller than previous mathematical programming algorithms. It scales up to large data sets and tree ensembles, where it provides, within seconds, systematic explanations grounded on well-defined models solved to optimality.
This talk corresponds to a recent work, accepted at ICML2021, and also accessible at https://arxiv.org/abs/2106.06631
November 1, 2021, 16.30 – 17.30 (CET)
Heuristics for Mixed-Integer Optimization through a Machine Learning Lens
To attend the seminar, click here.
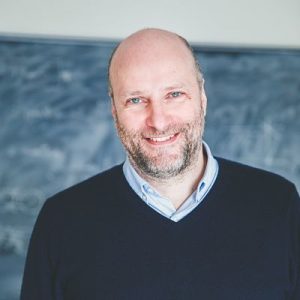
Speaker: Prof Andrea Lodi
Andrew H. and Ann R. Tisch Professor
Jacobs Technion-Cornell Institute
Cornell University
USA
Abstract: In this talk, we discuss how a careful use of Machine Learning concepts can have an impact in primal heuristics for Mixed-Integer Programming (MIP). More precisely, we consider two applications. First, we design a data-driven scheduler for running both diving and large-neighborhood search heuristics in SCIP, one of the most effective open-source MIP solvers. Second, we incorporate a major learning component into Local Branching, one of the most well-known primal heuristic paradigms. In both cases, computational results show solid improvements over the state of the art.
November 8, 2021, 16.30 – 17.30 (CET)
Using location-allocation optimisation to support specialised children’s ambulance services in England and Wales
To attend the seminar, click here.
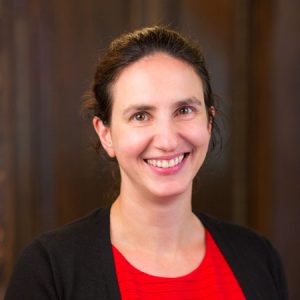
Abstract: Special paediatric intensive care retrieval teams (PICRTs), based in 11 locations across England and Wales, have been used to transport sick children from district general hospitals to one of 24 paediatric intensive care units since 1997. We worked with clinical teams to develop a location allocation optimisation framework to help inform decisions on the optimal number of locations for each PICRT, where those locations should be, which local hospital each location serves and how many teams should station each location. Our framework allows for stochastic journey times, differential weights for each journey leg and incorporates queuing theory by considering the time spent waiting for a PICRT to become available. We examine the average waiting time and the average time to bedside under different number of operational PICRT stations, different number of teams per station and different levels of demand and evaluate the current allocations across different demand scenarios.
November 15, 2021, 16.30 – 17.30 (CET)
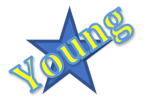
To attend the seminars, click here.
Improving the interpretability and fairness of (Generalized) linear models with categorical predictors
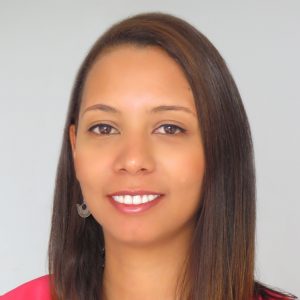
Abstract: We propose methodologies to reduce the complexity and improve the fairness of Generalized Linear Models in the presence of categorical predictors. The traditional one-hot encoding, where each category is represented by a dummy variable, can be wasteful, difficult to interpret, and prone to overfitting, especially when dealing with high-cardinality categorical predictors. In this talk, we address these challenges by finding a reduced representation of the categorical predictors by clustering their categories. This is done through a numerical method which aims to preserve (or even, improve) accuracy, while reducing the number of coefficients to be estimated for the categorical predictors. Our methodology can be extended to reduce unfairness in classification, i.e., the disparate mistreatment that a group of people sharing a sensitive attribute, such as race or religion, may be exposed to. In this case, we choose a reduced representation for the categorical features and the numerical features guided by the tradeoff between accuracy and disparate mistreatment. We illustrate the performance of our methods in real-world datasets.
A Mathematical Programming Approach to Sparse Canonical Correlation Analysis
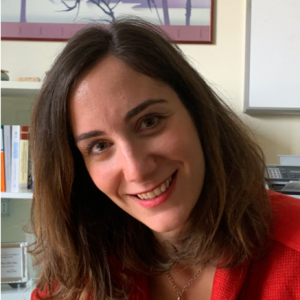
Speaker 2: Dr Lavinia Amorosi
Assistant Professor
Department of Statistical Sciences
University of Rome Sapienza
Italy
Abstract: In this talk we tackle Canonical Correlation Analysis (CCA), a dimensionality reduction method that summarises multiple data sources jointly, retaining their dependency structure. We propose a new technique to encode Sparsity in CCA by means of a new mathematical programming formulation which allows to obtain an exact solution using readily available solvers (like Gurobi). We show a preliminary investigation of the performance of our proposal through a simulation study, which highlights the potential of our approach.
This is a joint work with Tullia Padellini, from School of Public Health, Imperial College London.
See technical report at https://www.dss.uniroma1.it/it/system/files/pubblicazioni/A_Mathematical_Programming_Approach_to_Sparse_Canonical_Correlation_Analysis_final.pdf
Effects of Imbalanced Datasets on Interpretable Machine Learning
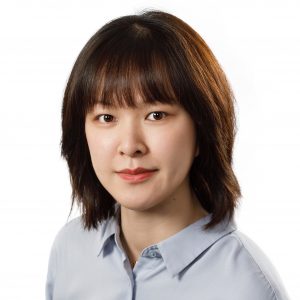
Speaker 3: Yujia Chen
PhD student
University of Edinburgh
UK
Abstract: In recent years, interpretability of machine learning techniques has drawn increasing attention in the credit scoring literature. Regulations have also made interpretability a clear requirement for the loan approval process. Therefore, many interpretation methods such as SHapley Additive exPlanations (SHAP) and Local Interpretable Model-agnostic Explanations (LIME), have been proposed to satisfy this requirement. These methods are used at a second stage to explain the prediction results generated by the ‘black-box’ machine learning models, which can keep the exceptional predictive accuracy of the machine learning models but also make the prediction interpretable.
Besides the interpretability problem, the class imbalance is also a concern in the credit scoring context, as the number of Bads is usually much less than the number of Goods in the credit scoring datasets. Current research has mainly focused on the effect of imbalanced datasets on predictive ability of machine learning techniques and how to improve it, while the impact on interpretability has never been studied before in the literature. Suppose the robustness of interpretation methods could be disturbed by the class imbalance problem – relying on the results generated by the less robust interpretation methods to classify the Goods and Bads can bring substantial financial loss to the financial institutions, and it will also pass the misleading information to the borrowers. This research conducts an empirical study using UK residential mortgage data to analyse how interpretable machine learning methods are affected by imbalanced credit scoring datasets, and the results show that the interpretations generated by some interpretation methods such as SHAP are less robust as the imbalance of the datasets increases
November 22, 2021, 17.30 – 18.30 (CET)
Stochastic Oracles and Where to Find Them
To attend the seminar, click here.
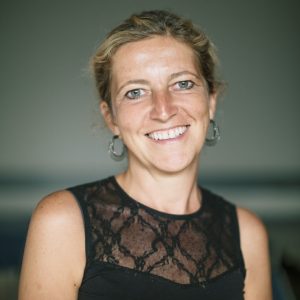
Speaker: Prof Katya Scheinberg
Professor and Director of Graduate Studies
Operations Research and Information Engineering
Cornell University
USA
Abstract: Continuous optimization is a mature field, which has recently undergone major expansion and change. One of the key new directions is the development of methods that do not require exact information about the objective function. Nevertheless, the majority of these methods, from stochastic gradient descent to «zero-th order» methods use some kind of approximate first order information. We will overview different methods of obtaining this information in different settings, including simple stochastic gradient via sampling, traditional and randomized finite difference methods and more. We will discuss what key properties of these inexact, stochastic first order oracles are useful for convergence analysis of optimization methods that use them.
November 29, 2021, 16.30 – 17.30 (CET)
Bored by Simple Numbers? Discriminant Analysis of Distributional Data
To attend the seminar, click here.
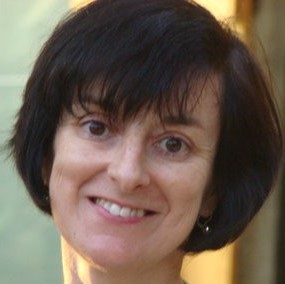
Abstract: In classical Statistics and Multivariate Data Analysis data is usually represented in a data array where each row represents a statistical unit, or “individual”, for which one single value is recorded for each variable (in columns). This representation model is however too restricted when the data to be analysed comprises variability. That is the case when the entities under analysis are not single elements, but groups formed e.g. based on some given common properties, and the observed variability within each group should be taken into account. To this aim, new variable types have been introduced, whose realizations are not single real values or categories, but sets, intervals, or distributions over a given domain. Symbolic Data Analysis provides a framework for the representation and analysis of such complex data, taking into account their inherent variability.
In this talk, we address classification of distributional data, where units are described by histogram or interval-valued variables. The proposed approach uses a linear discriminant function where distributions or intervals are represented by quantile functions, under specific assumptions. The identification of the corresponding model requires solving a specific optimization problem. The discriminant function allows defining a score for each unit, in the form of a quantile function, which is used to classify the units in two a priori groups, using the Mallows distance. An application of the proposed methodology will be presented.
This is a joint work with Sónia Dias, from ESTG – Polytechnic Institute of Viana do Castelo & LIAAD-INESC TEC (Portugal), and Paula Amaral, from CMA & FCT, NOVA University of Lisbon (Portugal).
December 6, 2021, 16.30 – 17.30 (CET)
AI and the 5th Industrial Revolution
To attend the seminar, click here.
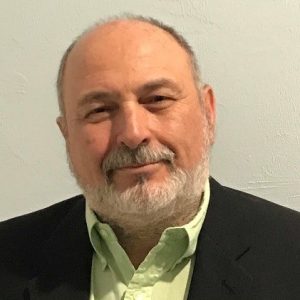
Speaker: : Prof Panos M. Pardalos
Paul and Heidi Brown Preeminent Professor in Industrial & Systems Engineering
University of Florida
USA
Abstract: Advances in machine learning/data sciences and AI are progressing rapidly and demonstrating the potential to transform our lives.
The spectacular success of these areas relies in part on their sophisticated mathematical underpinnings (e.g. optimization techniques and operations research tools), even though this crucial aspect is often downplayed. AI is on the cusp of the 5th Industrial Revolution.
In this lecture we will discuss progress from our perspective in the field of AI and its applications in Finance, Economics, Energy, Biomedicine and Smart Manufacturing.
December 13, 2021, 16.30 – 17.30 (CET)
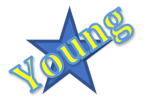
To attend the seminars, click here.
SOS-SDP: an Exact Solver for Minimum Sum-of-Squares Clustering
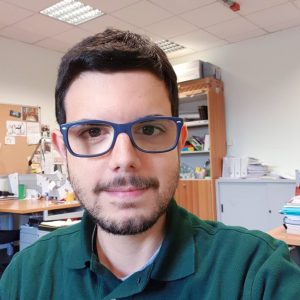
Abstract: Clustering is a fundamental tool in machine learning and data mining applications which takes many forms depending on the considered objective. One of the most popular formulations for clustering is the so-called Minimum Sum-of-Squares Clustering (MSSC): it aims to partition $n$ observations into $k$ clusters so that the sum of squared distances from each data point to the cluster center is minimized. Due to the NP-hardness of the MSSC, existing global optimization algorithms are capable of solving only small-scale instances. In this talk, we introduce SOS-SDP, an exact solver based
on the branch-and-bound technique. For the lower bound procedure, we solve the Semidefinite Programming (SDP) relaxation of the MSSC discrete optimization model and we use a cutting-plane procedure for strengthening the bound. For the upper bound computation, we exploit the solution of the SDP for a smart initialization of $k$-means algorithm, which is known to be sensitive to the choice of the initial centroids. Finally, our way of branching allows to reduce the size of the problem while going down the branch-and-bound tree. The obtained results show that SOS-SDP can successfully solve real-world instances with up to 4000 data points and with more than 20000 features.
See the paper at: https://arxiv.org/abs/2104.11542
Job shop and the train dispatching via deep reinforcement learning
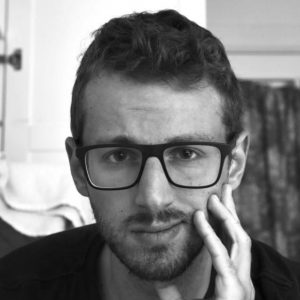
Speaker 2: Dr. Giorgio Grani
Research Scientist
SINTEF Digital
Norway
Abstract: Scheduling problems have been one of the most challenging and studied problems in optimization in the last decades, and researchers from all over the world have tried a large and varied set of methods. This talk will tackle the job shop scheduling problem and the train dispatching problem via deep reinforcement learning. In particular, we will exploit the power of actor-critic algorithms and flexible neural structures, like LSTM and graph convolutional networks. For the job shop, we fight against CPLEX, whereas for the train dispatching we compare it to other learning-based heuristics.
December 20, 2021, 16.30 – 17.30 (CET))
Challenges and improvements in optimization for machine learning
To attend the seminar, click here.
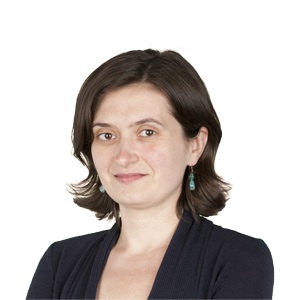
Abstract: We will discuss some key challenges to optimization algorithm development arising from machine learning. In particular, we investigate dimensionality reduction techniques in the variable/parameter domain for local and global optimization; these rely crucially on random projections (and hence connect to the seminar of L. Liberti in the previous week). We describe and use sketching results that allow efficient projections to low dimensions while preserving using properties, as well as other useful tools from random matrix theory and conic integral geometry. We focus on functions with low effective dimensionality – that are conjectured to provide an insightful proxy for neural networks landscapes. Time permitting we also discuss first- versus second-order optimization methods for training, and/or stochastic variants of classical optimization methods that allow biased noise, adaptive parameters and have almost sure convergence.
This work is joint with Jari Fowkes, Estelle Massart, Adilet Otemissov, Alex Puiu, Zhen Shao (Oxford University).
Talks of Season I
January 11, 2021, 16.30-17.30 (CET)
Rule Generation for Learning and Interpretation
To attend the seminar, click here.
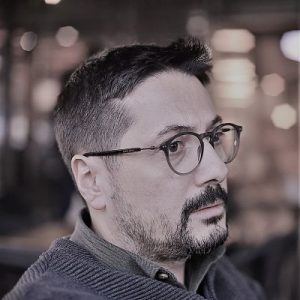
Speaker: Prof Ilker Birbil
Endowed Professor for the Chair in Data Science and Optimization.
Erasmus University Rotterdam The Netherlands.
Abstract: This talk consists of two parts. In the first part, we give a brief overview of rule-based learning algorithms using optimization and discuss each algorithm in terms of its prediction accuracy and interpretability. The second part of the talk is reserved for our recent work on a new rule learning algorithm. Our approach starts with modeling the learning process as a linear program, where the columns correspond to rules. Then we apply a column generation procedure to produce a set of rules. We discuss the difficulty of the proposed rule generation scheme and present a generic framework that can efficiently overcome this difficulty. With our approach, we observe that rule structure can be fine-tuned so that both accuracy and interpretability are considered simultaneously. Our suggested algorithm is capable of handling both classification and regression instances, and achieves accurate and interpretable results on various datasets from the literature.
January 18, 2021, 16.30-17.30 (CET)
Challenges in Fraud Analytics
To attend the seminar, click here.
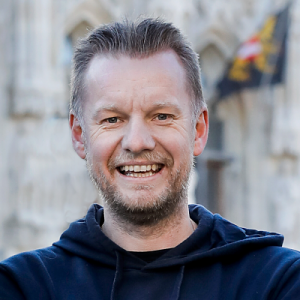
Speaker: Prof Bart Baesens
Professor of Big Data & Analytics KU Leuven. Belgium.
Abstract: In earlier work, we defined fraud as an uncommon, well-considered, imperceptibly concealed, time-evolving and often carefully organized crime which appears in many types and forms. In this presentation, we first elaborate on the uncommon and carefully organized elements of this definition. More specifically, we discuss the importance of sampling schemes and social networks in the building of analytical fraud models. Next, we introduce CS-logit or cost-sensitive logistic regression and illustrate how it can be used for detecting fraud. Throughout the presentation, we extensively embed our recent research findings on the topic.
January 25, 2021, 16.30 – 17.30 (CET)
Using Optimization to remove barriers for Machine Learning Applications in Power Systems
To attend the seminar, click here.
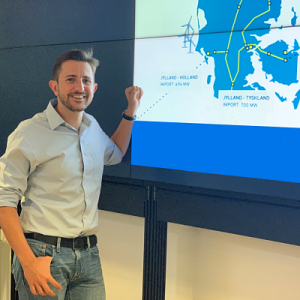
Speaker: Dr Spyros Chatzivasileiadis
Associate Professor at DTU Center for Electric Power and Energy DTU. Denmark.
Abstract: In this talk, we introduce methods that remove the barrier for applying neural networks in real-life power systems, and unlock a series of new applications. More specifically, we introduce a framework for (i) verifying neural network behavior in power systems and (ii) obtain provable worst-case guarantees of their performance. Up to this moment, neural networks have been applied in power systems as a black-box; this has presented a major barrier for their adoption in practice. Using a rigorous framework based on mixed integer linear programming, our methods can determine the range of inputs that neural networks classify as safe or unsafe; and, when it comes to regression neural networks, our methods allow to obtain provable worst-case guarantees of the neural network performance. Such methods have the potential to build the missing trust of power system operators on neural networks, and unlock a series of new applications in power systems and other safety-critical systems.
February 1, 2021, 16.30 – 17.00 (CET)
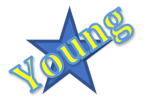
To attend the seminars, click here
Mathematical optimization in classification and regression trees
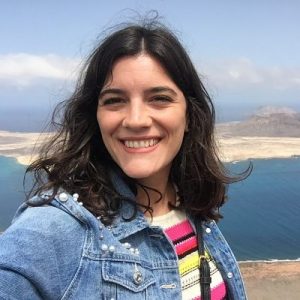
Speaker 1: Cristina Molero del Río
PhD student
IMUS-Instituto de Matemáticas de la Universidad de Sevilla. Spain.
Abstract: Classification and regression trees, as well as their variants, are off-the-shelf methods in Machine Learning. In this paper, we review recent contributions within the Continuous Optimization and the Mixed-Integer Linear Optimization paradigms to develop novel formulations in this research area. We compare those in terms of the nature of the decision variables and the constraints required, as well as the optimization algorithms proposed. We illustrate how these powerful formulations enhance the flexibility of tree models, being better suited to incorporate desirable properties such as cost-sensitivity, explainability and fairness, and to deal with complex data, such as functional data.
See paper at
Feature selection in nonlinear SVM using a novel min-max approach
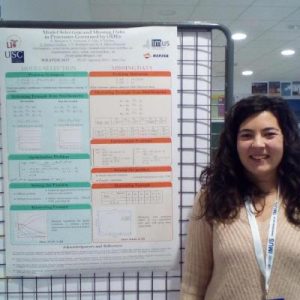
Speaker 2: Dr Asunción Jiménez Cordero
Postdoctoral Researcher
Research group Optimization and Analytics for Sustainable energY Systems (OASYS)
Universidad de Málaga. Spain.
Abstract: In recent years, feature selection has become a challenging problem in several machine learning fields, such as classification problems. Support Vector Machine (SVM) is a well-known technique applied in classification tasks. Various methodologies have been proposed in the literature to select the most relevant features in SVM. Unfortunately, all of them either deal with the feature selection problem in the linear classification setting or propose ad-hoc approaches that are difficult to implement in practice. In contrast, we propose an embedded feature selection method based on a min-max optimization problem, where a trade-off between model complexity and classification accuracy is sought. By leveraging duality theory, we equivalently reformulate the min-max problem and solve it without further ado using off-the-shelf software for nonlinear optimization. The efficiency and usefulness of our approach are tested on several benchmark data sets in terms of accuracy, number of selected features and interpretability.
See paper at
https://www.sciencedirect.com/science/article/abs/pii/S0377221720310195
Fair and Interpretable Decision Rules for Binary Classification
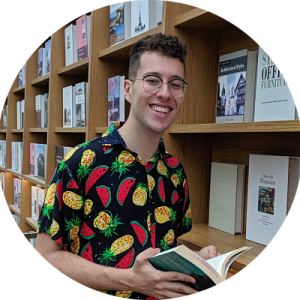
Abstract: In recent years, machine learning has begun automating decision making in fields as varied as college admissions, credit lending, and criminal sentencing. The socially-sensitive nature of some of these applications together with increasing regulatory constraints has necessitated the need for algorithms that are both fair and interpretable. In this talk I’ll discuss the problem of building Boolean rule sets in disjunctive normal form (DNF), an interpretable model for binary classification, subject to explicit fairness constraints. We formulate the problem as an integer program that maximizes classification accuracy with explicit constraints on two different measures of classification parity: equality of opportunity, and equalized odds. A column generation framework, with a novel formulation, is used to efficiently search over exponentially many possible rules, eliminating the need for heuristic rule mining. When combined with faster heuristics, our method can deal with large data-sets.
February 8, 2021, 16.30 – 17.30 (CET)
Optimization of Optimal Sparse Decision Trees
To attend the seminar, click here.
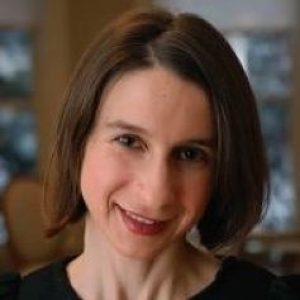
Speaker: Prof Cynthia Rudin
Professor of Computer Science, Electrical and Computer Engineering, and Statistical Science.
Duke University. USA.
Abstract: With widespread use of machine learning, there have been serious societal consequences from using black box models for high-stakes decisions, including flawed bail and parole decisions in criminal justice, flawed models in healthcare, and black box loan decisions in finance. Transparency and interpretability of machine learning models is critical in high stakes decisions. In this talk, I will focus on a fundamental and important problem in the field of interpretable machine learning: optimal sparse decision trees. We would like to find trees that maximize accuracy and minimize the number of leaves in the tree (sparsity). This is an NP hard optimization problem with no polynomial time approximation. I will present the first practical algorithm for solving this problem, which uses a highly customized dynamic-programming-with-bounds procedure, computational reuse, specialized data structures, analytical bounds, and bit-vector computations. We can sometimes produce provably optimal sparse trees in about the same amount of time that CART produces a (non-optimal, greedy) decision tree. See paper at
https://arxiv.org/abs/2006.08690.
February 15, 2021, 16.30 – 17.30 (CET)
Location Analysis meets Data Science
To attend the seminar, click here.
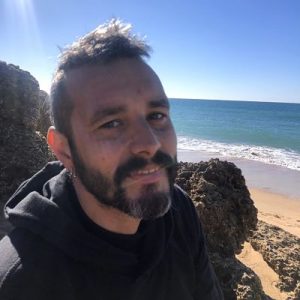
Abstract: Location Analysis deals with the problems of determining the «optimal» position of services in order to satisfy the demand of a set of customers. Most of the applications in this field usually come from Network Design or Logistics, but the mathematical nature of these problems goes beyond these applications. In particular, the most popular mathematical optimization based approaches for supervised classification can be seen as particular versions of location problems with adequate selections of the set of users and the facilities that are desired to be located. This connection allows one to exploit the modeling and algorithmic power of some of the widely studied problems in Facility Location to be applied to derive novel supervised learning tools. We illustrate this synergy between the two fields providing a general framework to estimate multivariate linear regression and multisource-regression models, extending SVM-based classification approaches to different metrics, and deriving novel multiclass classification tools.
February 22, 2021, 16.30 – 17.30 (CET)
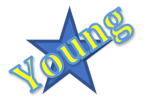
To attend the seminar, click here.
Clustering and Interpreting via Mathematical Optimization
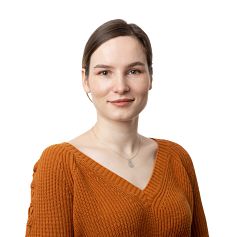
Abstract: Explainability is nowadays a must. Researchers and practitioners need to interpret the results of black-box machine learning models for, e.g., model selection as well legal and ethical purposes. In this paper, we introduce an optimization model to make cluster analysis more interpretable. Clusters may be already given, and thus our aim is to find rule-based explanations for them. Alternatively, we can simultaneously define the clusters and choose the explanations, thus clustering is guided by a dissimilarity measure and an interpretability measure as well. More precisely, we aim to separate individuals and choose explanations as accurately as possible by a mathematical optimization model with three objectives: minimization of the sum of squared dissimilarities for each pair of individuals belonging to the same cluster, maximization of the total number of true positive cases (i.e., the number of individuals in a cluster that satisfy the explanations chosen for the cluster), and minimization of the total number of false positive cases (i.e., the number of individuals outside the cluster that satisfy the explanations chosen for the cluster). We propose as solution approach an alternating procedure: do clustering when explanations are chosen, and interpret when clusters are fixed. We illustrate our approach on several well-known datasets with different sizes and types of data.
Binary Classification via Ellipsoidal Separation
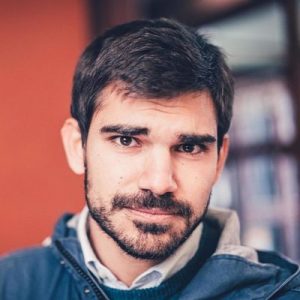
Speaker 2: Dr Benedetto Manca
Postdoctoral Researcher
Dipartimento di Matematica e Informatica
Università di Cagliari. Italy.
Abstract: Given two finite point sets X and Y in an Euclidean space, we consider two different separation criteria for X and Y using an ellipsoid S. In the first approach we construct the minimum volume ellipsoid containing X and not containing Y. We model this problem starting from the SVM model with quadratic kernel imposing the fact that the hyperplane in the feature space projects onto an ellipsoid in the input space.
In the second approach we construct the minimum volume ellipsoid which intersects every segment connecting points of X with points of Y. The corresponding problem is non-convex and we we solve it heuristically via an iterative algorithm of the Gauss-Seidel type solving alternatively an SDP program and a quadratically constrained (convex) program. Starting from these two separation criteria we develop a binary classification criteria. Some preliminary numerical results are presented.
Adaptive Hyper-box Matching for Interpretable Individualized Treatment Effect Estimation
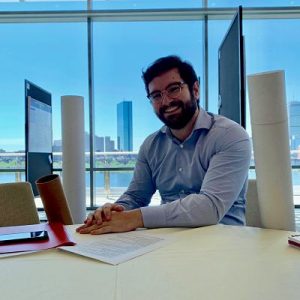
Abstract: We propose a matching method for observational data that matches units with others in unit-specific, hyper-box-shaped regions of the covariate space. These regions are large enough that many matches are created for each unit and small enough that the treatment effect is roughly constant throughout. The regions are found as either the solution to a mixed integer program, or using a (fast) approximation algorithm. The result is an interpretable and tailored estimate of a causal effect for each unit.
See paper at https://arxiv.org/abs/2003.01805
March 1, 2021, 10.00 – 11.00 (CET)
"Improving" prediction of human behavior using behavior modification
To attend the seminar, click here.
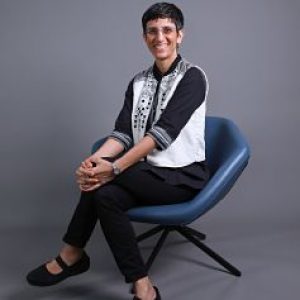
Speaker: Prof Galit Shmueli
Editor, INFORMS Journal on Data Science
Tsing Hua Distinguished Professor and Institute Director
Institute of Service Science, College of Technology Management
National Tsing Hua University Taiwan
Abstract: The fields of machine learning and statistics have invested great efforts into designing algorithms, models, and approaches that better predict future observations. Larger and richer data have also been shown to improve predictive power. This is especially true in the world of human behavioral big data, as is evident from recent advances in behavioral prediction technology. Large internet platforms that collect behavioral big data predict user behavior for their internal commercial purposes as well as for third parties, such as advertisers, insurers, security forces, and political consulting firms, who utilize the predictions for user-level personalization, targeting, and other decision-making. While machine learning algorithmic and data efforts are directed at improving predicted values, the internet platforms can minimize prediction error by «pushing» users’ actions towards their predicted values using behavior modification techniques. The better the platform is able to make users conform to their predicted outcomes, the more it can boast both its predictive accuracy and its ability to induce behavior change. Hence, internet platforms have a strong incentive to «make the prediction true», that is, demonstrate small prediction error. This strategy is absent from the machine learning and statistics literature. Investigating the properties of this strategy requires incorporating causal terminology and notation into the correlation-based predictive environment. However, such an integration is currently lacking. To tackle this void, we integrate Pearl’s causal do(.) operator to represent and integrate intentional behavior modification into the correlation-based predictive framework. We then derive the Expected Prediction Error given behavior modification, and identify the components impacting predictive power. Our formulation and derivation make transparent the impact and implications of such behavior modification to data scientists, internet platforms and their clients, and importantly, to the humans whose behavior is manipulated. Behavior modification can make users’ behavior not only more predictable but also more homogeneous; yet this apparent predictability is not guaranteed to generalize when the predictions are used by platform clients outside of the platform environment. Outcomes pushed towards their predictions can also be at odds with the client’s intention, and harmful to the manipulated users.
March 8, 2021, 16.30 – 17.30 (CET)
Cost-sensitive causal classification: novel methodologies and application in business analytics
To attend the seminar, click here.
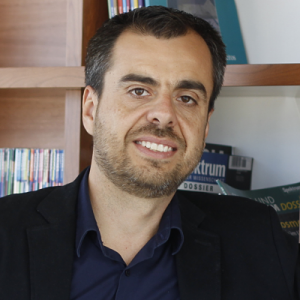
Abstract: Churn prediction is a well-known business analytics task whose goal is to identify customers that are likely to leave a company voluntarily. Once potential churners are detected, a retention campaign is performed for enhancing customer loyalty. This is extremely beneficial for customers since engaged customers generate more revenue than other clients, it reduces operational costs, and it avoids the misspending of money caused by inefficient marketing campaigns. In this talk, we address the churn prediction task via cost-sensitive and causal classification. On the one hand, we present a novel profit-driven machine learning method: the Profit-based Minimax Probability Machine (ProfMPM). This method maximizes the profit of a retention campaign directly in the objective function using a robust optimization setting. On the other hand, we discuss novel churn prediction applications via cost-sensitive and causal classification, such as the mutual fund industry and student dropout in higher education. This talk concludes with the foundations of cost-sensitive causal classification, a novel research topic that combines both worlds and we believe it has an enormous potential in business analytics and other tasks.
March 15, 2021, 16.30 – 17.30 (CET)
Ethical ML: mind the assumptions
To attend the seminar, click here.
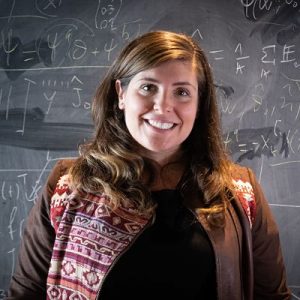
Speaker: Prof Isabel Valera
Saarland University and Max Planck Institute for Software System. Germany.
Abstract: As automated data analysis supplements and even replaces human supervision in consequential decision-making (e.g., pretrial bail and loan approval), there are growing concerns from civil organizations, governments, and researchers about potential unfairness and lack of transparency of these algorithmic systems. To address these concerns, the emerging field of ethical machine learning has focused on proposing definitions and mechanisms to ensure the fairness and explicability of the outcomes of these systems. However, as we will discuss in this work, existing solutions are still far from being perfect and encounter significant technical challenges. Specifically, I will show that, in order for ethical ML, it is essential to have a holistic view of the system, starting from the data collection process before training, all the way to the deployment of the system in the real-world. Wrong technical assumptions may indeed come at a high social cost.
As an example, I will first focus on my recent work on both fair algorithmic decision-making, and algorithmic recourse. In particular, I will show that algorithms may indeed amply the existing unfairness level in the data, if their assumptions do not hold in practice. Then, I will focus on algorithmic recourse, which aims to guide individuals affected by an algorithmic decision system on how to achieve the desired outcome. In this context, I will discuss the inherent limitations of counterfactual explanations, and argue for a shift of paradigm from recourse via nearest counterfactual explanations to recourse through interventions, which directly accounts for the underlying causal structure in the data. Finally, we will then discuss how to achieve recourse in practice when only limited causal information is available.
March 22, 2021, 16.30 – 17.30 (CET)
Contextual decision-making under uncertainty
To attend the seminar, click here.
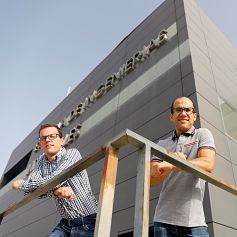
Speakers:
Dr Juan Miguel Morales González
Associate Professor.Research Group Optimization and Analytics for Sustainable energY Systems (OASYS).Universidad de Málaga.Spain
&
Associate Professor.Research Group Optimization and Analytics for Sustainable energY Systems (OASYS).Universidad de Málaga.Spain.
Abstract: The world is witnessing an unprecedented explosion in the amount of information, in the form of data, observations and measurements, about physical and social processes and systems. The exponential growth of the amount of data available has brought about new opportunities in the field of Optimization-based Decision Making. Of all of them, in this talk, we will explore the possibility of using this data to formulate optimization models that allow making decisions with a higher level of confidence about their practical performance. For this purpose, we assume that the data at the decision maker’s disposal correspond to measurements or observations of processes that together form a potentially predictive or explanatory context of the factors that directly affect the decision to be made. Then, to exploit this context for improving decision making, we will propose both a parametric and a non-parametric approach. The former uses Bilevel Programming to build predictive models tailored to the decision-making process, while the latter utilizes Distributionally Robust Optimization to infer the decision directly from the contextual data. Throughout this talk, we will illustrate both approaches using examples from inventory management, portfolio optimization and strategic offering in electricity markets.
April 12, 2021, 16.30 – 17.30 (CET)
Trespassing Random Forests with a pointed stick for self defence
To attend the seminar, click here.
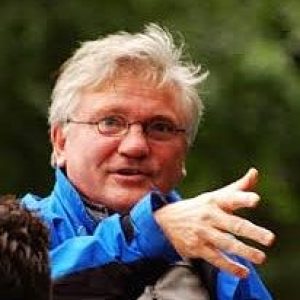
Speaker: Prof Wolfgang Härdle
Ladislaus von Bortkiewicz Professor of Statistics School of Business and Economics
Humboldt-Universität zu Berlin. Germany.
Abstract: We give a tour through some random forests (RF) and, review mathematical approaches and present some old Huberizing ideas. RFs are data dependent smoothers that evade partially the curse of dimensionality since they locally adapt by suppressing non informative features. A la limite, RFs obey to Chuck Stone’s speed limits, though.
April 19, 2021, 16.30 – 17.30 (CET)
Partition-based formulations for mixed-integer optimization of trained ReLU neural networks
To attend the seminar, click here.
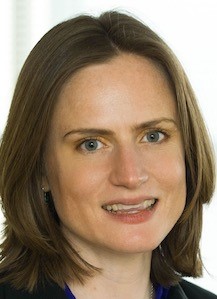
Speaker: Prof Ruth Misener
Imperial College London.UK.
This work develops a class of relaxations in between the big-M and convex hull formulations of disjunctions, drawing advantages from both. We show that this class leads to mixed-integer formulations for trained ReLU neural networks. The approach balances model size and tightness by partitioning node inputs into a number of groups and forming the convex hull over the partitions via disjunctive programming. At one extreme, one partition per input recovers the convex hull of a node, i.e., the tightest possible formulation for each node. For fewer partitions, we develop smaller relaxations that approximate the convex hull, and show that they outperform existing formulations. Specifically, we propose strategies for partitioning variables based on theoretical motivations and validate these strategies using extensive computational experiments. Furthermore, the proposed scheme complements known algorithmic approaches, e.g., optimization-based bound tightening captures dependencies within a partition.
This joint work with Calvin Tsay, Jan Kronqvist, Alexander Thebelt is based on two papers: https://arxiv.org/abs/2102.04373 and https://arxiv.org/abs/2101.12708.
April 26, 2021, 16.30 – 17.30 (CET)
Integer optimization for predictive and prescriptive analytics in high stakes domains
To attend the seminar, click here.
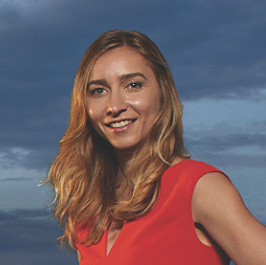
Speaker: Dr Phebe Vayanos
Assistant Professor of Industrial & Systems Engineering and Computer Science
Associate Director of CAIS Center for Artificial Intelligence in Society
University of Southern California. USA.
Abstract: Data-driven predictive and prescriptive analytics tools are increasingly being used to assist decision-making in high stakes domains (e.g., to prioritize people experiencing homelessness for scarce housing resources, to identify individuals at risk of suicide, and to design public health interventions). The deployment of such algorithms in these domains that can impact people’s lives and societal outcomes creates an urgent need for algorithms that are fair and interpretable and that leverage the available data to its full extent to yield the most accurate decisions. In this presentation, we discuss our recent works that leverage tools from integer optimization and causal inference to design optimal, interpretable, and fair decision-support tools that are suitable to deploy in high stakes settings
May 3, 2021, 16.30 – 17.30 (CET)
Block-and-Sample Decomposition in Deep Network Training
To attend the seminar, click here.
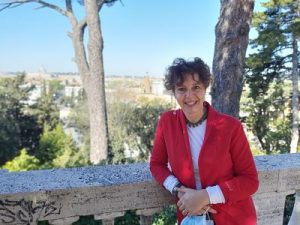
Speaker: Prof Laura Palagi
Department of Computer, Control and Management Engineering
Sapienza University of Rome.Italy.
Abstract: The talk focuses on block coordinate decomposition methods when optimizating a finite sum of functions. Specifically, we focus on methods for training Deep Feedforward Neural Networks (DFNNs). We present new ideas combining online (mini batch) methods and block-coordinate decomposition that can exploit the layered structure of a deep network. Indeed, a clever decomposition may lead to efficient calculations and simpler problem to be solved. We analyse convergence properties and report numerical results proving effectiveness of the approach when applied to train Deep Networks on a benchmark test set. In particular, the focus is on whether such techniques can lead to better solutions (e.g. avoiding local minima) and reduce the amount of time needed for the optimisation process.
This is joint work with Ruggiero Seccia.
May 10, 2021, 16.30 – 17.30 (CET)
Optimize to learn to optimize: the Algorithm Configuration Problem
To attend the seminar, click here.
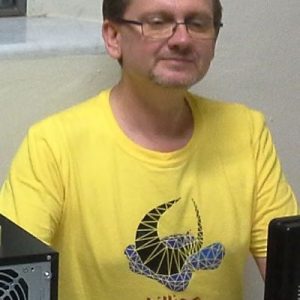
Abstract: Given a problem (P) and a parametrised algorithm A for solving instances of (P), the Algorithm Configuration Problem requires to find the parameters configuration C of A yielding the highest performance p(I;C) of A for solving a given instance I of (P).
Thus, ACP is an optimization problem on the known set F of feasible configurations; however, usually no analytic form of its objective p(I;.) is known. Therefore, it is natural to try to learn an approximation p_M() of p() by some Machine Learning approach, and then use p_M(I;.) as the objective of the ACP. Most approaches doing so in the literature treat p_M() as a black-box and use the corresponding methods. However, these methods are known not to scale well as the number of parameters in C increases, which is always the case with complex algorithms having tens or hundreds of them.
We take a different stance, whereby we exploit the mathematical structure of the ML approach underlying p_M to formulate the ACP as a (typically, mixed-integer and nonlinear) mathematical program on F. Thus, we can solve it with all the available sophisticated machinery, and even off-the-shelf optimization tools, hopefully scaling more efficiently to large search spaces F. Our approach is in general applicable to diverse ML approaches such as logistic regression, support vector regression, neural networks, decision trees, etc. We will quickly review the relevant literature, point out the several nontrivial issues that need to be overcome, and present some computational experiments on the solution of hard combinatorial optimization problems by means of a general-purpose MILP solver.
May 17, 2021, 16.30 – 17.30 (CET)
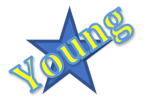
To attend the seminars, click here.
A unified approach to Counterfactual Explanations by means of Mathematical Optimization
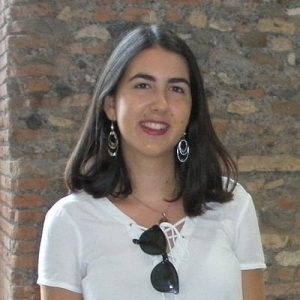
Abstract: Due to the increasing use of complex machine learning models, it has become more and more important to be able to understand and explain their behaviour. An effective class of post-hoc explanations are counterfactual explanations, i.e. minimal perturbations of the predictor variables to change the prediction for a specific instance. In this talk, we will provide a multi-objective mathematical formulation for different state-of-the-art models used for the prediction, specifically applied for multi-class classification models. A running example with real-world data will be used to illustrate our method.
Optimize to learn to optimize: getting down and dirty
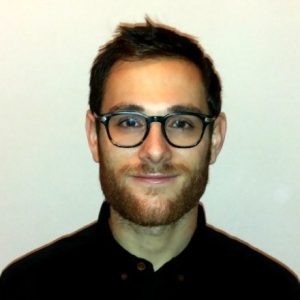
Speaker 2: Gabriele Iommazzo
PhD student LIX-École Polytechnique
Institut Polytechnique de Paris.France.
Abstract: Given a problem (P) and an algorithm A for solving the instances of (P), the Algorithm Configuration Problem (ACP) consists of finding the parameter configuration C of A allowing the highest algorithmic performance p(I;C) on a specific instance I of (P). Thus, the ACP can be formulated as an optimization problem, where the constraints define the set F of feasible configurations, and the objective optimizes the performance function p.
Since p is usually a black-box function (i.e., its analytic form is unknown), the ACP methodology we devised first learns an approximation M of p by Machine Learning (ML); then, it formulates the ACP as a Mathematical Program (MP), whose objective optimizes M and whose constraints define F. This MP is solved, upon the arrival of a new instance I, to retrieve the algorithmic configuration C* achieving optimal estimated performance M(I;C*); C* is, hopefully, a good configuration for actually solving I with A.
The novelty of our approach lies in the fact that, since we treat the ACP as an MP, we can exploit the exact form of the MP to use existing advanced solution algorithms; these algorithms are expected to be more efficient on large F than the black-box optimization methods, prominent in the literature. Our approach can be adapted to work with many ML algorithms, as long as one is capable of translating the mathematical properties underlying M into MP terms.
We will present several versions of our approach, for M corresponding to different, well-regarded ML methodologies, and compare their computational performances for tuning the parameters of an optimization solver, run on instances of a hard mixed-integer linear programming problem. In particular, we will investigate how implementation choices in the learning phase affect not only the accuracy of M, but also the cost of solving the derived MP, yielding nontrivial trade-offs
End-to-end decision-focussed learning over combinatorial problems
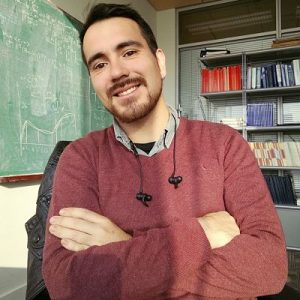
Speaker 3: Dr Victor Bucarey López
Data Analytics Lab
Vrije Universiteit Brussel. Belgium.
Abstract: Many decision-making processes involve solving a combinatorial optimization problem with uncertain input that can be estimated from historic data. In this talk we show the main algorithmic challenges involved in the process of learning a decision-task oriented cost vector for combinatorial optimization. In particular, we show its relationship with bilevel optimization and what are the different techniques proposed in the literature to find these vectors via an end-to-end learning procedure.
May 24, 2021, 16.30 – 17.30 (CET)
The Max-Cut Decision Tree: Improving on the Accuracy and Running Time of Decision Trees and Random Forests
To attend the seminar, click here.
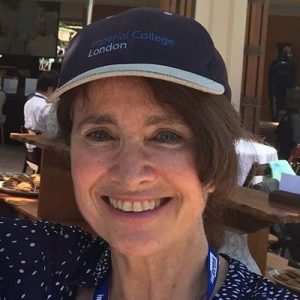
Speaker 1: Prof Dorit S. Hochbaum
Chancellor Full Professor
Department of Industrial Engineering and Operations Research
University of California, Berkeley.USA.
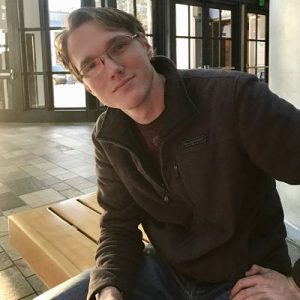
Speaker 2: Jon Bodine
Department of Industrial Engineering and Operations Research
University of California, Berkeley. USA.
Abstract: Decision trees are a widely used method for classification, both alone and as the building blocks of multiple different ensemble learning methods. The Max-Cut decision tree involves novel modifications to a standard, baseline model of classification decision tree, precisely CART Gini. One modification involves an alternative splitting metric, Maximum Cut, which is based on maximizing the distance between all pairs of observations that belong to separate classes and separate sides of the threshold value. The other modification is to select the decision feature from a linear combination of the input features constructed using Principal Component Analysis (PCA) locally at each node. Our experiments show that this node-based, localized PCA with the novel splitting modification can dramatically improve classification, while also significantly decreasing computational time compared to the baseline decision tree. Moreover, our results are most significant when evaluated on data sets with higher dimensions, or more classes. For the example data set CIFAR-100, the modifications enabled a 49% improvement in accuracy, relative to CART Gini, while reducing CPU time by 94% for comparable implementations. These introduced modifications are expected to dramatically advance the capabilities of decision trees for difficult classification tasks. The benefits of using the Max-Cut Decision trees are demonstrated to extend to random forests as shown in synthetic experimentation to date.
June 7, 2021, 16.30 – 17.30 (CET)
Diffusion Asymptotics for Sequential Experiments
To attend the seminar, click here.
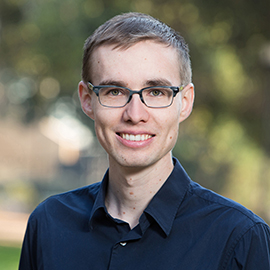
Speaker: Dr Stefan Wager
Assistant Professor of Operations, Information, and Technology.
Stanford Graduate School of Business. USA.
Abstract: We propose a new diffusion-asymptotic analysis for sequentially randomized experiments. Rather than taking sample size n to infinity while keeping the problem parameters fixed, we let the mean signal level scale to the order 1/√n so as to preserve the difficulty of the learning task as n gets large. In this regime, we show that the behavior of a class of methods for sequential experimentation converges to a diffusion limit. This connection enables us to make sharp performance predictions and obtain new insights on the behavior of Thompson sampling. Our diffusion asymptotics also help resolve a discrepancy between the Θ(log(n)) regret predicted by the fixed-parameter, large-sample asymptotics on the one hand, and the Θ(√n) regret from worst-case, finite-sample analysis on the other, suggesting that it is an appropriate asymptotic regime for understanding practical large-scale sequential experiments.
See paper at https://arxiv.org/abs/2101.09855.